I just became curious about whether or not a service similar to Digg but not dealing with independent tags but rather with MOM edges .. networks of tags, done in Rails, might become a self-sufficient starter for MOM. People could plug-in recognizers, reorganizers, network downloaders, network merging tools by themselves.
Approaching that this way, I'd have something presentable already (also presentable to myself), plus I'd get some relieve from the pressure to get the whole framework out, soon. -- I just applied for a new job, and that one almost does not leave me any air to breathe. So, everything related to MOM might shift out quite a while -- I've got Christmas 2008 already on my "radar screen".
However, mainly drafted for 2008, at least, is to get some scientific rock-solid articles out, dealing with matters related to MOM.
Updates: none so far
Content Representation With A Twist
Thursday, December 27, 2007
Thursday, October 25, 2007
text/editor auto-completion as a possible real world application for MOM
Right now, I am using my secondary workplace PC. At this one, I am used to use it one-handedly. And let the auto-completion kick in.
In a recent blog posting somewhere else, I was discussing lectures, lecturers, discussing as a topic, and the next issue I moved to was seminars. Intuitively, I expected, the auto-completion would kick in and offer "seminars" -- which it didn't.
I pondered whether to file a feature request, suggesting to background-use a thesaurus -- a word-processing one, not necessarily a real one -- to predict the words one might most-likely use soon. -- Then, I nticed, traditional term ordering systems like e.g. thesauri might have a hard time to do so; even more the programmers who actually should implement such kind of tool... well, on the second glance, maybe brute force could help there, and as a text is a relatively small amount of data (and vocabularies even more small), might be doable, easily to implement.
The brute force approach could pick up, stem the words of the text, then follow all the relation edges of a term to its set of neighbours, collect them, order them by alphabet, consider them like the words appearing really i the text: offer them for auto-completion where it looks appropriately.
On the other hand, a MOM approach might be to consider the words of the already typed-in text, step back a step, see the features of the items of the terms, count which other item(s) count the most features the until-now mentioned ones feature too. That way, we additionally would get a ranking of probability of upcoming terms. ... I'd do that myself, but the issue on tasks like this remans the old one: Where to get such interrelated collections of words in a reasonable amount and for reasonable .. no cost at all?
Updates: none so far
In a recent blog posting somewhere else, I was discussing lectures, lecturers, discussing as a topic, and the next issue I moved to was seminars. Intuitively, I expected, the auto-completion would kick in and offer "seminars" -- which it didn't.
I pondered whether to file a feature request, suggesting to background-use a thesaurus -- a word-processing one, not necessarily a real one -- to predict the words one might most-likely use soon. -- Then, I nticed, traditional term ordering systems like e.g. thesauri might have a hard time to do so; even more the programmers who actually should implement such kind of tool... well, on the second glance, maybe brute force could help there, and as a text is a relatively small amount of data (and vocabularies even more small), might be doable, easily to implement.
The brute force approach could pick up, stem the words of the text, then follow all the relation edges of a term to its set of neighbours, collect them, order them by alphabet, consider them like the words appearing really i the text: offer them for auto-completion where it looks appropriately.
On the other hand, a MOM approach might be to consider the words of the already typed-in text, step back a step, see the features of the items of the terms, count which other item(s) count the most features the until-now mentioned ones feature too. That way, we additionally would get a ranking of probability of upcoming terms. ... I'd do that myself, but the issue on tasks like this remans the old one: Where to get such interrelated collections of words in a reasonable amount and for reasonable .. no cost at all?
Updates: none so far
Tuesday, October 23, 2007
adjusting the direction of this blog: blogging on current neuro issues
I've been working on the Model of Meaning "ideas conglomerate" since more than seven years now. The first question I count to be part of that system of ideas I asked in summer 2000, during a more or less boring lesson on some economics subject.
Unfortunately, I picked up the issue before I became introduced into the methodology of working scientifically. So what I figured out, what I read, what I observed, perceived went into a big mix-up. Which brought me into some trouble: Since I apprehend several issues of behaviour, perception, neurology/thinking each a while before someone else published their papers on the issue -- I read about them in a popular science magazine -- I strongly believe, I am right with my course throught the complex. However, I started without sticking to scientific methods, but I figured out things. -- To gain the reputation ("credits"), I thought I should get for that work, I had to put the whole building of what I've figured out onto a new, stable, scientific foundation. But the same time, I already felt unable to differenciate between what I figured out by myself and what I learnt from any external source: What someone was telling might or might not imply what I figured out already. How to make sure, they and me meant, implied the same?
To prove, I were right, I thought the better opportunity would be to just implement the whole idea as a piece of software -- that is what you know by MOM today.
However, as I am unemployed currently, I became really distracted from the MOM project. And involved in more professional blogging. Which continuously carries along the question, how to increase one's reputation.
Now, I was reading a posting of a not so reliable popular science [kind of] blog on sleep deprivation, how it'd affect rational thinking. As sleep is a topic I touched by MOM several times, I was interested in verifying whether or not the "blog" was re-narrating correctly. As CiteSeer seems to be down, currently, I launched Google Scholar with a demand for articles of Seung-Schik Yoo for 2007. (In the hope to get the article.) However, accidentally, I found A deficit in the ability to form new human memories without sleep by the same person (co-author), published in February 2007. Which nudged me even further to my insights gained by MOM. -- As I am currently experiencing a regular visitor from Korea on this MOM blog, I thought it might be worth a shot to start just blogging about MOM -- even if I don't have any scientific reputation in that field of topic.
That's why you are reading this posting here.
The impulse was, I might gain and convince some audience, maybe even gain some reputation in this field of topic, despite not any scientific one. However, I think, it might become some fun to comment on what's going on in this area, even without any scientific degree here.
Additionally, I am interested in perception, usability, comprehensibility, everything that has anything to do with mind and memory. But one thing, I am not interested in. That is artificial intelligence. When I touched intelligence any time in the past, it was a by-product at all.
Whatever. Let's see whether or not it'd actually blog on it...
Updates: none so far
Unfortunately, I picked up the issue before I became introduced into the methodology of working scientifically. So what I figured out, what I read, what I observed, perceived went into a big mix-up. Which brought me into some trouble: Since I apprehend several issues of behaviour, perception, neurology/thinking each a while before someone else published their papers on the issue -- I read about them in a popular science magazine -- I strongly believe, I am right with my course throught the complex. However, I started without sticking to scientific methods, but I figured out things. -- To gain the reputation ("credits"), I thought I should get for that work, I had to put the whole building of what I've figured out onto a new, stable, scientific foundation. But the same time, I already felt unable to differenciate between what I figured out by myself and what I learnt from any external source: What someone was telling might or might not imply what I figured out already. How to make sure, they and me meant, implied the same?
To prove, I were right, I thought the better opportunity would be to just implement the whole idea as a piece of software -- that is what you know by MOM today.
However, as I am unemployed currently, I became really distracted from the MOM project. And involved in more professional blogging. Which continuously carries along the question, how to increase one's reputation.
Now, I was reading a posting of a not so reliable popular science [kind of] blog on sleep deprivation, how it'd affect rational thinking. As sleep is a topic I touched by MOM several times, I was interested in verifying whether or not the "blog" was re-narrating correctly. As CiteSeer seems to be down, currently, I launched Google Scholar with a demand for articles of Seung-Schik Yoo for 2007. (In the hope to get the article.) However, accidentally, I found A deficit in the ability to form new human memories without sleep by the same person (co-author), published in February 2007. Which nudged me even further to my insights gained by MOM. -- As I am currently experiencing a regular visitor from Korea on this MOM blog, I thought it might be worth a shot to start just blogging about MOM -- even if I don't have any scientific reputation in that field of topic.
That's why you are reading this posting here.
The impulse was, I might gain and convince some audience, maybe even gain some reputation in this field of topic, despite not any scientific one. However, I think, it might become some fun to comment on what's going on in this area, even without any scientific degree here.
Additionally, I am interested in perception, usability, comprehensibility, everything that has anything to do with mind and memory. But one thing, I am not interested in. That is artificial intelligence. When I touched intelligence any time in the past, it was a by-product at all.
Whatever. Let's see whether or not it'd actually blog on it...
Updates: none so far
Tuesday, October 09, 2007
on using tags in file system
Stumbled upon, but not yet read. It`s a 2005 blog entry of anyone on using tags in file systems.
Updates: none so far
Updates: none so far
Monday, August 20, 2007
removed: Sidebar element "Objective"
Objective
Common quality of today's information technology, in an aim to become able to identify items, is to mark up every single item. – The Model of Meaning heads to build the foundation to manage-without any such markup.
The approach is about content representation in the literal sense of the term.
Updates: none so far
Common quality of today's information technology, in an aim to become able to identify items, is to mark up every single item. – The Model of Meaning heads to build the foundation to manage-without any such markup.
The approach is about content representation in the literal sense of the term.
Updates: none so far
Friday, August 10, 2007
Search results on graph algorithms
I asked before, whether or not someone might be interested in improving the kind N network detection algorithm. -- Well, I figured, I "shot first, asked then", figuratively, implemented the approach before asking Google about the issue. Hm. Foolish.
But, as I grasped the idea now, that's a chance for further improvement of the detection approach. However, I want to get that baby implemented once in complete before I go into any source improving. Hence, I keep the offer: If you're interested in diving into MOM, the source, want to improve it, its source, or especially the kind N network detection, please let me know. I'd be curiously to hear from you.
Updates: none so far
But, as I grasped the idea now, that's a chance for further improvement of the detection approach. However, I want to get that baby implemented once in complete before I go into any source improving. Hence, I keep the offer: If you're interested in diving into MOM, the source, want to improve it, its source, or especially the kind N network detection, please let me know. I'd be curiously to hear from you.
Updates: none so far
Detecting kind N networks: Speed comparison of matrix and graph based approaches
The approach of determining kind N networks I took a year before -- when I was still using Perl and drafting the approach by functional programming [which later became hardly to comprehend] -- was to mark all the edges into a matrix and detect rectangular, non-intersecting, virtual areas there.
Virtual areas in a matrix? If you consider a non-zero cell of a matrix to be a spot of an area, a larger such area constitutes by adjacent spots (non-zero cells). I consider them being rectangular when they cover an area of at least 2 x 2 cells of the matrix, better: at least 2 x 3 or 3 x 2. A 2 x 3 area equals the W network (3 x 2 is the M network; W and M networks both are kind N networks). Fine, so far. But virtual?
There might be lines of adjacent spots within the matrix, but the lines might be away from another line of spots, i.e. not adjacently. But some rows or columns away. These lines, together, although being disjacent, can get considered to constitute an area -- a virtual. That's because the x and y values represent a node each, hence the non-zero cells within the matrix are edges. There's really no need that the node which was set up to be column x to be fixed at that position. Hence, the columns -- and rows -- of the matrix are freely swappable. In other words, we shift around the marked spots within the matrix to get a real area.
See the picture aside: There are blue, red and green marked cells. Obviously, the blue spots form an area, since they neighbour each other.
The lower part of the green area is a step more complicated: We could get the 2 x 3 area, if we'd ignored the upper part of the green, by simply swapping columns 4 and 1. To get the remainder of the green, we need to swap rows 2 and 5. Which, of course would disrupt the blue area.
However, we could note down the blue area first and swap for the green area afterwards,
The red area, then, is the most complex one, at first glance, but after swapping around a bit, it gets found as well -- yet rather simply.
As the non-zero cells within the matrix are edges, a 10 x 10 matrix as a whole could contain up to a hundred different edges, i.e. get and be densely filled.
But there's a restriction with the matrix, not visible in the diagram: The columns and the rows represent the same nodes. So, as the MOM graph allows no loops, less than half of the matrix may be filled, actually, -- the upper right half of the matrix less the diagonal from top left to bottom right. So, in reality, the matrix never gets really dense.
However, I implemented the approach -- and while it worked fine with a matrix as small as 10 x 10 or 20 x 20, when I launched examination of a 1000 x 1000 matrix I quickly became aware, that approach might be "a bit" slow: It took hours on a 2 GHz machine (single core x86 CPU). Actually, the necessary processing time increased exponentially. -- And a thousand nodes is really not that much. Really, not even worth to mention: Just think about the number of words being part of a common day's news feed.
Well, now I developed another approach of detecting kind N networks within a MOM network, doing it by considering the edges only. That, effectively, leaves out the white spaces of the matrix.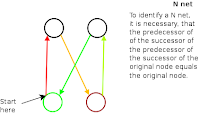
As before, I started with a relatively small net -- a hundred nodes and about 200 .. 250 edges. Which did it in less than half a minute, on a 400 MHz machine (single core CPU). Launching a 1000 nodes large test net with 2,500 to 5,000 edges, I learned it took about an hour. -- I became a bit scared because of that development, but then figured the reason for that slowness might be that it's just a 400 MHz machine only.
I put it onto the before mentioned 2000 MHz computer. -- The about 5000 edges got examined in less but three minutes. -- Phew!.
But I think, there are chances to speed up the approach, still. Anyone interested in improving the code or algorithm?
But, yes, sadly, I didn't check in the code yet, since I am after implementing detecting and replacing [the found] kind N networks. And the latter part I didn't figure out yet.
Updates: none so far
Virtual areas in a matrix? If you consider a non-zero cell of a matrix to be a spot of an area, a larger such area constitutes by adjacent spots (non-zero cells). I consider them being rectangular when they cover an area of at least 2 x 2 cells of the matrix, better: at least 2 x 3 or 3 x 2. A 2 x 3 area equals the W network (3 x 2 is the M network; W and M networks both are kind N networks). Fine, so far. But virtual?
There might be lines of adjacent spots within the matrix, but the lines might be away from another line of spots, i.e. not adjacently. But some rows or columns away. These lines, together, although being disjacent, can get considered to constitute an area -- a virtual. That's because the x and y values represent a node each, hence the non-zero cells within the matrix are edges. There's really no need that the node which was set up to be column x to be fixed at that position. Hence, the columns -- and rows -- of the matrix are freely swappable. In other words, we shift around the marked spots within the matrix to get a real area.

See the picture aside: There are blue, red and green marked cells. Obviously, the blue spots form an area, since they neighbour each other.
The lower part of the green area is a step more complicated: We could get the 2 x 3 area, if we'd ignored the upper part of the green, by simply swapping columns 4 and 1. To get the remainder of the green, we need to swap rows 2 and 5. Which, of course would disrupt the blue area.
However, we could note down the blue area first and swap for the green area afterwards,
The red area, then, is the most complex one, at first glance, but after swapping around a bit, it gets found as well -- yet rather simply.
As the non-zero cells within the matrix are edges, a 10 x 10 matrix as a whole could contain up to a hundred different edges, i.e. get and be densely filled.
But there's a restriction with the matrix, not visible in the diagram: The columns and the rows represent the same nodes. So, as the MOM graph allows no loops, less than half of the matrix may be filled, actually, -- the upper right half of the matrix less the diagonal from top left to bottom right. So, in reality, the matrix never gets really dense.
However, I implemented the approach -- and while it worked fine with a matrix as small as 10 x 10 or 20 x 20, when I launched examination of a 1000 x 1000 matrix I quickly became aware, that approach might be "a bit" slow: It took hours on a 2 GHz machine (single core x86 CPU). Actually, the necessary processing time increased exponentially. -- And a thousand nodes is really not that much. Really, not even worth to mention: Just think about the number of words being part of a common day's news feed.
Well, now I developed another approach of detecting kind N networks within a MOM network, doing it by considering the edges only. That, effectively, leaves out the white spaces of the matrix.
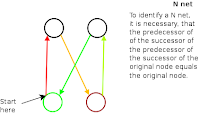
As before, I started with a relatively small net -- a hundred nodes and about 200 .. 250 edges. Which did it in less than half a minute, on a 400 MHz machine (single core CPU). Launching a 1000 nodes large test net with 2,500 to 5,000 edges, I learned it took about an hour. -- I became a bit scared because of that development, but then figured the reason for that slowness might be that it's just a 400 MHz machine only.
I put it onto the before mentioned 2000 MHz computer. -- The about 5000 edges got examined in less but three minutes. -- Phew!.
But I think, there are chances to speed up the approach, still. Anyone interested in improving the code or algorithm?
But, yes, sadly, I didn't check in the code yet, since I am after implementing detecting and replacing [the found] kind N networks. And the latter part I didn't figure out yet.
Updates: none so far
Thursday, August 09, 2007
How does a question get stored? Does an answer replace a question? How do we find out that there's a chance to get a question answered?
One day amidst my course of studies, I wondered about how questions (i.e. question texts) and answers might get stored in mind. That curiousity was the first step to the later Model of Meaning, nowadays also known as Content Representation model. I wondered whether an answer might replace a question one day:
To mention context together with a question applies meaning to the question. When the question gets answered, the anwer might accompany that meaning. -- Well, wenn the question is answered thoroughly, I think, the answer might replace the question.
Does that mean, that in mind the question gets stored as a placeholder for any upcoming answer? How long does any such placeholder [if it is such a one] hold that place before it gets replace [if it gets replaced at all] by the/an answer?
Or does mind set up any data node that tells "lack of information"? And the question gets generated instantly? -- And if so, does that generation take place when there's indeed a chance to get the question answered? But what might be the trigger for finding out that there's a chance to get the question answered?
Labels:
20000512.11-42h CET,
content storing,
context,
meaning,
on answers,
on questions
Benefits of detecting and replacing kind N networks: revealing implied content
Added documentation to the sub-framework of detection of replacable partitial networks, and rewrote parts of initialization for a few classes. Actually, what I am talking here about is the kind N networks detection.
Kind N networks, in the MOM terminology, are such ones of four or more nodes, two predecessors (cf. image: nodes A and B), two successors (nodes C and D), and each of the predecessors connected to each of the successors. That looks like a mixture of an "N" and an "X" character.
As the X often gets used to indicate something unknown, but here is not anything unknown, that kind of network got called the N network.
-- Add a pair of successor and predecessor, and it still looks somewhat like that X-N mixture. As it features more but two base and two top points, we call it a pure kind N network: any MOM sub-network that consists of an equal number of predecessor and successor nodes (and wires all the predecessor to the successor nodes) is called a pure kind N network. Thus, the N network, of course, is also a pure kind N network.
Then, there are chances, a network features more successor nodes but predecessor ones.
If the number of predecessor nodes is >= 2, that kind of net gets called a kind W network, because of its shape. -- It gets called the W network, if it sports only two predecessor and exactly three successor nodes.
If a graph features only a single predecessor node, it's a kind V fan. Similar to the naming scheme for the W network, a kind V fan gets called the V fan, if its shape matches the letter: If it features a single predecessor and exactly two successor nodes.
Put upside down, we get a kind M network, the M network, a kind A fan and the A fan, resepectively.
Because kind W/M networks follow the pure kind N network approach by wiring each predecessor with each successor node, all together -- kind W, kind M and pure kind N networks get summarized under the generic "kind N network" label.
So, the efforts done were to detect any kind N networks within a larger MOM network. Why? -- The complete wiring of each predecessor node with each successor node results in a situation,
that the content of each successor node equals the content of all predecessor nodes together (plus the content of any separate predecessor nodes the successors don't share with their neighbours of that kind N network). The image aside shows it: Nodes F and G share the content of B, C and D. A becomes part of F only, so does E for G. -- That sharing of common predecessor nodes implies two things:
Now, part of it was rewritten and all of it documented. Now that sub-framework for kind N net detection needs to get spread into separate class files and put into a sub-directory or sub-directory hierarchy.
Updates: none so far

As the X often gets used to indicate something unknown, but here is not anything unknown, that kind of network got called the N network.

Then, there are chances, a network features more successor nodes but predecessor ones.

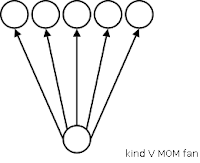
Put upside down, we get a kind M network, the M network, a kind A fan and the A fan, resepectively.
Because kind W/M networks follow the pure kind N network approach by wiring each predecessor with each successor node, all together -- kind W, kind M and pure kind N networks get summarized under the generic "kind N network" label.
So, the efforts done were to detect any kind N networks within a larger MOM network. Why? -- The complete wiring of each predecessor node with each successor node results in a situation,
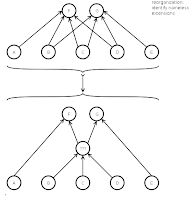
- First, as any MOM node represents the merged content of its predecessor nodes, we could replace the heavy wiring by adding a new node and wire link all predecessor nodes of the kind N network to that newly added node and it to all the successor nodes. That way the number of edges needed to administer could get reduced from a * b to only a + b.
- Second, as now it might be obvious, that wiring of all the predecessor nodes to each of the successor nodes was nothing different but an implication. A not explied (?)/explicited (?) notion. By adding the node, we make it explicit.
Now, part of it was rewritten and all of it documented. Now that sub-framework for kind N net detection needs to get spread into separate class files and put into a sub-directory or sub-directory hierarchy.
Updates: none so far
Tuesday, July 31, 2007
Finished a first piece of reorganization
Just finished: One core part of reorganization -- finding large replacable partitial networks. I figured, that might get me rid of those double feed news, as having this functionality available might enable me to sort news by topic. ... Which makes me ponder about wrapping this bit into a rails site. ;-)
Updates: none so far
Updates: none so far
Friday, July 27, 2007
chance for a MOM application: get old news filtered from RSS feeds
Development on the MOM SSC framework and especially implementing one core part of the reorganizer got lagged because I am still after getting a job (and other issues). Apparently, that search distracts more but actually having a job.
However, the time to read the feeds I defend. But there I found a problem -- too much interesting news and too many repetitions of the same topic. I survived one Apple keynote time, and I endured the Vista market introduction. But when there was another hype on the iPhone I begun feeling nagged.
Now, as the iPhone wave gets prolonged by iPhone hacks, and as noone can hid from that Harry Potter hype, I really get annoyed. -- As the Model of Meaning provides the logic to detect similarities, I want a tool that determines old news and variants of yet known news. Such as the latest iPhone hack or Potter p2p share.
Another way but looking up and dealing with the tags of feed entries, might be to take the words of any set of two or more articles and see for sets of words they share. A more brute-force (and less MOM way approach would be to take word neighbourhoods (word sequences) into consideration. -- On the other hand, the tool-to-be could use wordnet to include synonyms into 'consideration' when looking for similarities between texts.
For that reason, now I see how I can get through with the beforementioned reorganizer core -- the one that actually detects similarities for to save edges, i.e. storage -- logical by edges as well as "physically" by disk space.
Updates: 20070731: linked the word "lagged" to the last recent release posting
However, the time to read the feeds I defend. But there I found a problem -- too much interesting news and too many repetitions of the same topic. I survived one Apple keynote time, and I endured the Vista market introduction. But when there was another hype on the iPhone I begun feeling nagged.
Now, as the iPhone wave gets prolonged by iPhone hacks, and as noone can hid from that Harry Potter hype, I really get annoyed. -- As the Model of Meaning provides the logic to detect similarities, I want a tool that determines old news and variants of yet known news. Such as the latest iPhone hack or Potter p2p share.
Another way but looking up and dealing with the tags of feed entries, might be to take the words of any set of two or more articles and see for sets of words they share. A more brute-force (and less MOM way approach would be to take word neighbourhoods (word sequences) into consideration. -- On the other hand, the tool-to-be could use wordnet to include synonyms into 'consideration' when looking for similarities between texts.
For that reason, now I see how I can get through with the beforementioned reorganizer core -- the one that actually detects similarities for to save edges, i.e. storage -- logical by edges as well as "physically" by disk space.
Updates: 20070731: linked the word "lagged" to the last recent release posting
Friday, July 13, 2007
Positive hits in the "content representation" search results
Correct hits on the "content representation" term Google search (in opposite to any such hits that contained "content <something else but whitespace only, such as punctuation> representation"):
The above results I picked from pages 79 and 78 only -- and already learned a lession: It might make more sense to apply some kind of clustering here instead of walking through the list manually. Even the intellectual check whether there is anything in between of "content" and "representation" -- to filter out false hits --, can be done by software.
I'd like to learn the most-often used terms (besides of "content representation"), and, by help of that clustering/visualization, I want to get the chance to ignore obvious false hits.
That demands for using -- get hands on -- the Google API.
Updates: none so far
- Mining Web Documents for Unintended Information Revelation
- LHNCBC: Mansur Project - Anatomical Text to Images
- Data processing: presentation processing of document patents new
- Philippe Jost - LTS2
- nformation Sciences Institute - Research
- [PDF] Music notation/representation requirements for the protection of ...
- [PDF] Microsoft PowerPoint - Research_GET_STIC ASIE.ppt
- Informed Content Delivery Across Adaptive Overlay Networks
- Digital Television Applications
- [PDF] Abstract Model, 70–76 Accessibility characteristics, 262 Act, 217 ...
- [PS] Selecting Task-Relevant Sources for Just-in-Time Retrieval
- [Cc-uk] FW: IEE Events] The 2nd European Workshop on the ...
- [PDF] T R D R
- XML Pitstop : Largest Source of XML Examples on the Web
- ...
The above results I picked from pages 79 and 78 only -- and already learned a lession: It might make more sense to apply some kind of clustering here instead of walking through the list manually. Even the intellectual check whether there is anything in between of "content" and "representation" -- to filter out false hits --, can be done by software.
I'd like to learn the most-often used terms (besides of "content representation"), and, by help of that clustering/visualization, I want to get the chance to ignore obvious false hits.
That demands for using -- get hands on -- the Google API.
Updates: none so far
wanted: tag cloud for the other pages mentioning the term "content representation"
I'd like to learn what all these 91,900 search results related to content representation might be about. (Curiously, I wonder where I left the article directly pointing to that search result -- when it still were 88,900 "only".)
To learn that quickly, first I need to decide whether to see the pages manually or "mechanically". Then, I'd need to learn how to use the Google API to quickly get all the hits -- which actually end by page 78 which in fact is not 90 thousand plus search results but only a "small" number of only 788 hits.
However, since I'd like to redo this search every now and then again, and as I might like to do the search for sites like Cite Seer as well, it might be worth the effort to develop a small program which helps me in determining the content of all the pages. -- A tag cloud and toying around with precision and recall might contribute a bit to the visualized cloud. -- The cloud terms' sizes could visualize quantity in recall, while the precision might get indicated by color incoding, e.g. blue .. green .. yellow .. orange .. red, like on maps, where high precision might get indicated by red and low precision by blue.
There's a tag cloud generator available in Debian's share of Perl libraries. I already modified it, and it's available on demand. -- However, I'd prefer to have any place in the web to put my version to. Any repository out there for that library?
Updates: none so far
To learn that quickly, first I need to decide whether to see the pages manually or "mechanically". Then, I'd need to learn how to use the Google API to quickly get all the hits -- which actually end by page 78 which in fact is not 90 thousand plus search results but only a "small" number of only 788 hits.
However, since I'd like to redo this search every now and then again, and as I might like to do the search for sites like Cite Seer as well, it might be worth the effort to develop a small program which helps me in determining the content of all the pages. -- A tag cloud and toying around with precision and recall might contribute a bit to the visualized cloud. -- The cloud terms' sizes could visualize quantity in recall, while the precision might get indicated by color incoding, e.g. blue .. green .. yellow .. orange .. red, like on maps, where high precision might get indicated by red and low precision by blue.
There's a tag cloud generator available in Debian's share of Perl libraries. I already modified it, and it's available on demand. -- However, I'd prefer to have any place in the web to put my version to. Any repository out there for that library?
Updates: none so far
A hunger for analysis and play(ing), after cramming data into one's memory
After having speed-read a book of project management, my mind starves for any analytical task to do. Not necessarily of the stuff just read/learnt but of anything.
Might it be possible that being confronted with a bold set of news results in a bold number of newly available neurons -- that kind-of want/need to bee stored somewhere, get wired in somewhere/any better in case they' are already wired in, somewhat? Does that task urge, since it might feel unpleasant otherwise?
— Indeed, the motivation behind that hunger for analysis, in fact, might be to give (any) thing a trial, to experiment.
Updates: none so far
Might it be possible that being confronted with a bold set of news results in a bold number of newly available neurons -- that kind-of want/need to bee stored somewhere, get wired in somewhere/any better in case they' are already wired in, somewhat? Does that task urge, since it might feel unpleasant otherwise?
— Indeed, the motivation behind that hunger for analysis, in fact, might be to give (any) thing a trial, to experiment.
Updates: none so far
Thursday, July 12, 2007
Every beginning is hard.
The interesting question about "Every beginning is hard." is: Why? -- Not: For what reason, but by what origin? By what cause? What, on a neurologic basis is it that makes the beginnings so hard? Is there a way to overcome the beginnings to be hard, any way?
Updates: none so far
Updates: none so far
Sunday, July 01, 2007
other models of meaning
Maybe worth a skim: Search results on 'Model of Meaning'. ('Content Representation with a Twist' didn't find anything so far, neither on Google, nor on Yahoo. Although Yahoo's crawler visited the MOM development project page over at gna.org.)
Updates: none so far
Updates: none so far
Friday, June 29, 2007
New release of MOM SSC
New version is out!
Updates: none so far
- Now with test cases in place for all classes of the framework,
- heavily reworked HandledSet class and
- documentation for HandledSet too -- with a peek to the principles of reorganization.
Updates: none so far
Thursday, June 28, 2007
Why Do I Approach Developing MOM The Way Visible By MOM SSC?
The MOM Simple Set Core (MOM SSC) is the most recent implementation of MOM. MOM is a trinity of research, development and a project driving both of them ahead. In core, MOM is the Model of Meaning plus research based on that model, aiming at representing every kind of content bare of words and tagging, only based on graphs and bare input sensors, such as 'light given', 'oxygene here', 'soft ground'. -- However, since 'there is a red light under that passenger seat, calmly blinking' is a bit more complex content, and that content is not yet developed by graph, currently MOM accepts crutches -- labels or pieces of software that signal a certain event being given, e.g 'web browser cannot render that page correctly'. As MOM improves, such crutches shall get replaced by the more flexible (and error resistant) representation of content as offered by MOM.
There are several promised benefits of that. Getting content available without words implies the the chance to render content to any language of the world. Getting there without tagging implies the chance that the machine knows of the content represented -- instead of just dealing with it but remaining unaware of what it means. That in turn implies the chance to load content ("knowledge") into any sort of machines, such as traffic lights or vacuum cleaners or cars. Whereby to load the knowledge might be much a bit quicker but needing to train any sort of neuronal network AI. -- MOM is not after implementing any sorts of artificial intelligence but heads for getting the content available. Call it a [content-addressable] memory.
That error resistant representation of content beforementioned originates from another core part of MOM, the recognition. -- Yes, that's right. MOM found recognition to be a part of memory, not of any sorts of intelligence. It's an automatic process which, however, might be supportable by training [link: "is it learning?"]: weighting the graph's edges. [It's clear to me that humans can improve their recognition, but I am not sure whether the causes of learning equal those of improving the recognition abilities of a MOM net, hence the differentiation.] Core of MOM's recognition and cause for its error resistance is that while the MOM net defines every possible feature of an item, for recognition not every such one must be given, only a few. -- Which, by the way, matches a claim recently posted by Chris Chatham: Only a few of the features of a known item result in a correct recognition of that item because there are only the yet known items out there: To discern all the items being similar, you don't need that many different features. But wait the day you encounter an in fact new item! -- You'd get it wrong, in any case. Remember the days you were familar to dogs as the only kind of pet animals? Then, encountering the first pet cat, you likely named it 'dog', din't you? Same so for any kind of flip pictures, like the one you can either see a beautiful young woman in or a rather old one. -- To get back to Chatham: On the issue of change blindness he claimed "[...] the brain is 'offloading' its memory requirements to the environment in which it exists: why bother remembering the location of objects when a quick glance will suffice?"
Along with research, MOM is a project of development. I am used to program, hence cast MOM into software is the most clear way to go. MOM, casted to software, allows for verifying the model. Also, over time, a full implementation of MOM might result, hence achieve to get handy all the chances MOM offers.
For example, the MOM Simple Set Core (MOM SSC) originally was only after implementing the MOM net, i.e. the functionality to maintain (parts of) a MOM net in computer memory (RAM). That's overcome now. Now, going further ahead, MOM SSC aims at implementing the reorganizer. That's a share of MOM which shrinks the graph by kepping the same content -- yet even revealing content which was only implicit beforehand.
Former versions of MOM parts were implemented using Perl. For reasons of readability, for MOM SSC, Ruby was chosen. Since the theoretical work on the reorganizer it was clear, the reorganizer modifies the MOM net, hence challenges the strengths of the recognizer. To get able to make the recognizer perform well even on reorganized MOM nets, I now begun to implement the reorganizer. Having it in place, research on the recognition might go into depth. Especially since having a reorganizer in place implies to get enabled to automatically test quality of recognition: Recognition on the reorganized net should provide the same results as recognition performed on the original net. Fine part is, neither reorganization nor recognition need any labels for the nodes (i.e.: no mark-up/tagging).
Upcoming milestone of the MOM SSC sub-project might be to implement the core of the reorganizer, accompanied by full duck typing approach for the MOM SSC classes, or/and by fixing all the chances for improvement, which accumulated over time since the beginnings of MOM SSC. -- Core of the reorganizer is to detect and replace sub-networks of the MOM graph that occupy (far) more nodes/edges than necessary to represent a piece of content. The replace would be to reduce these sub-networks to just as many nodes/edges as actually needed to represent the content.
Updates: none so far
There are several promised benefits of that. Getting content available without words implies the the chance to render content to any language of the world. Getting there without tagging implies the chance that the machine knows of the content represented -- instead of just dealing with it but remaining unaware of what it means. That in turn implies the chance to load content ("knowledge") into any sort of machines, such as traffic lights or vacuum cleaners or cars. Whereby to load the knowledge might be much a bit quicker but needing to train any sort of neuronal network AI. -- MOM is not after implementing any sorts of artificial intelligence but heads for getting the content available. Call it a [content-addressable] memory.
That error resistant representation of content beforementioned originates from another core part of MOM, the recognition. -- Yes, that's right. MOM found recognition to be a part of memory, not of any sorts of intelligence. It's an automatic process which, however, might be supportable by training [link: "is it learning?"]: weighting the graph's edges. [It's clear to me that humans can improve their recognition, but I am not sure whether the causes of learning equal those of improving the recognition abilities of a MOM net, hence the differentiation.] Core of MOM's recognition and cause for its error resistance is that while the MOM net defines every possible feature of an item, for recognition not every such one must be given, only a few. -- Which, by the way, matches a claim recently posted by Chris Chatham: Only a few of the features of a known item result in a correct recognition of that item because there are only the yet known items out there: To discern all the items being similar, you don't need that many different features. But wait the day you encounter an in fact new item! -- You'd get it wrong, in any case. Remember the days you were familar to dogs as the only kind of pet animals? Then, encountering the first pet cat, you likely named it 'dog', din't you? Same so for any kind of flip pictures, like the one you can either see a beautiful young woman in or a rather old one. -- To get back to Chatham: On the issue of change blindness he claimed "[...] the brain is 'offloading' its memory requirements to the environment in which it exists: why bother remembering the location of objects when a quick glance will suffice?"
Along with research, MOM is a project of development. I am used to program, hence cast MOM into software is the most clear way to go. MOM, casted to software, allows for verifying the model. Also, over time, a full implementation of MOM might result, hence achieve to get handy all the chances MOM offers.
For example, the MOM Simple Set Core (MOM SSC) originally was only after implementing the MOM net, i.e. the functionality to maintain (parts of) a MOM net in computer memory (RAM). That's overcome now. Now, going further ahead, MOM SSC aims at implementing the reorganizer. That's a share of MOM which shrinks the graph by kepping the same content -- yet even revealing content which was only implicit beforehand.
Former versions of MOM parts were implemented using Perl. For reasons of readability, for MOM SSC, Ruby was chosen. Since the theoretical work on the reorganizer it was clear, the reorganizer modifies the MOM net, hence challenges the strengths of the recognizer. To get able to make the recognizer perform well even on reorganized MOM nets, I now begun to implement the reorganizer. Having it in place, research on the recognition might go into depth. Especially since having a reorganizer in place implies to get enabled to automatically test quality of recognition: Recognition on the reorganized net should provide the same results as recognition performed on the original net. Fine part is, neither reorganization nor recognition need any labels for the nodes (i.e.: no mark-up/tagging).
Upcoming milestone of the MOM SSC sub-project might be to implement the core of the reorganizer, accompanied by full duck typing approach for the MOM SSC classes, or/and by fixing all the chances for improvement, which accumulated over time since the beginnings of MOM SSC. -- Core of the reorganizer is to detect and replace sub-networks of the MOM graph that occupy (far) more nodes/edges than necessary to represent a piece of content. The replace would be to reduce these sub-networks to just as many nodes/edges as actually needed to represent the content.
Updates: none so far
Tuesday, June 26, 2007
Reorganizing Tags -- For What Benefit?
Having in sight to get over the core MOM reorganization obstacle and get reorganization implemented, as well as having noticed a possible benefit of having only//just//at least a reorganizer at hand (i.e. without any reorganizer) [aside of the benefit of becoming able to develop a more sophisticated recognizer then], I begun thinking about whether there might be a chance to make some profit by providing the MOM reorganizer as a web service.
Still unknowingly about any profitable such web service, I ended up with looking up 'tagging' in wikipedia. Which might be worth a read, same so for the German variant of that very article [for those of you comfortable with that language].
Updates: none so far
Still unknowingly about any profitable such web service, I ended up with looking up 'tagging' in wikipedia. Which might be worth a read, same so for the German variant of that very article [for those of you comfortable with that language].
Updates: none so far
Labels:
benefit,
business model,
folksonomy,
profit,
recognition,
reorganization,
tagging,
web service,
worth a read
Monday, June 25, 2007
homework to do: learn the vocabulary of neuro(-bio-)logy, provide reliable..rock-proof definitions
The recent posting on familiarity, recognition, creation of new neurons, their offshoot, self, and the brain causes me another set of things to do for a homework:
Updates: none so far
- Get my reliable definitions for the topics I am dealing with, here, online, publicly. Such as for neuron, axon, cell division, dendrite.
- Learn the proper vocabulary for the items I don't know by name, such as rank growth, what somebody is aware of, somewhat, knows that an item exists (if that's being called 'knowledge', that is rather too less discerning for my purposes), nerve cell core body and others.
Updates: none so far
questions regarding familiarity, recognition, creation of new neurons, their offshoot, self, and the brain
Saturday morning I awoke when I was scratching my head. I noticed the sound it made. I thought of something like -- Why does it make that sound it makes? That well known sound. Then I started to wonder -- which made me woke finally. Thinking is always a good setting [for me] to get ripped out of the sweetest (and the most horrible dreams), so this one was.
So, fine, scratching my head makes a sound. A familiar sound. One I know really good. Do I? That sound is so familiar I most often not even notice. -- That was what I noticed next: Why didn't I notice it so far? How many times may I have scratched my head up to now? And only now I ask that question. Curious.
Might it be that as soon as we are familiar to a situation/thing we stop asking further questions on that matter? Might this be the cause for why children [apparently] ask about everything? Is their familiarity [with the world] so sparse that recognition can not kick in? Or might it be, recognition itself results in too vague results [for the children]: i.e. results in 1..many nodes which get stimulated to a similar degree, thus automatic ("intuitive") recognizing, that results in a single most probable [represented] item recognized, cannot take place? Therefore, the child has to find that single most probable item consciously, actively? They support recognition by asking grown-ups? And by that support they make a distinct edges become weighted as more important? [I assume, that equals <learning>. The body is able to move a lid or a leg by a pulse of a nerve -- why not move or even grow a neuron's dendrite or axon by basic will?]
If the child, by the approach to weight single edges more important, does not achieve the wanted result, maybe because, after a while, all the edges get weighted equally again [hence the confusion gets as strong as when it was the time before weighting at all], what happens then? Does the child decide -- read: does the child decide, as well as: does the child decide -- one or more new neurons to create?
Or gets this decision made by "the brain"? Or does it cause the creation of new nerve cells without any kind of decision-making, i.e. automatically? Or is it just any single nerve cell which initiates cell division? Or is it not even that single neuron which 'initiates' cell division but plainly begins to divide itself, caused by any external conditions, e.g. biological or chemical ones, which in turn might get caused because there is an obviously needed nerve cell not in place? Might these biological or chemical conditions get caused because neighbouring cells feel some stress and excrete some hormones?
Or might be the reason for new neurons to be created be caused by any neuro biological condition, though? Maybe because nerve cells divide when any of their offshoots -- axons, dendrites -- grew a "too large" tree//braid//knop? And, this rank growth divides itself from the remainder of the very nerve cell?
Or might it be that at some time there's no place left over on the main body of a neuron where any other neurons immediately can dock to, hence dendrites get started to grow? Or the docking nerve cells begin to grow axons, since these might fit between all the other dockers? Or is it that way, the nerve cell gets divided when there's no place left over on the core body of it?
PS.: I doubt there is any bird's view instance which decides whether or not to set up any new edge or cell (node). In other words, I doubt "the brain" decides that..anything at all what takes place within the brain itself//brain body, i.e. I doubt there is any other instance in brain but 'self' that makes any decisions regarding brain itself.
Updates: none so far
So, fine, scratching my head makes a sound. A familiar sound. One I know really good. Do I? That sound is so familiar I most often not even notice. -- That was what I noticed next: Why didn't I notice it so far? How many times may I have scratched my head up to now? And only now I ask that question. Curious.
Might it be that as soon as we are familiar to a situation/thing we stop asking further questions on that matter? Might this be the cause for why children [apparently] ask about everything? Is their familiarity [with the world] so sparse that recognition can not kick in? Or might it be, recognition itself results in too vague results [for the children]: i.e. results in 1..many nodes which get stimulated to a similar degree, thus automatic ("intuitive") recognizing, that results in a single most probable [represented] item recognized, cannot take place? Therefore, the child has to find that single most probable item consciously, actively? They support recognition by asking grown-ups? And by that support they make a distinct edges become weighted as more important? [I assume, that equals <learning>. The body is able to move a lid or a leg by a pulse of a nerve -- why not move or even grow a neuron's dendrite or axon by basic will?]
If the child, by the approach to weight single edges more important, does not achieve the wanted result, maybe because, after a while, all the edges get weighted equally again [hence the confusion gets as strong as when it was the time before weighting at all], what happens then? Does the child decide -- read: does the child decide, as well as: does the child decide -- one or more new neurons to create?
Or gets this decision made by "the brain"? Or does it cause the creation of new nerve cells without any kind of decision-making, i.e. automatically? Or is it just any single nerve cell which initiates cell division? Or is it not even that single neuron which 'initiates' cell division but plainly begins to divide itself, caused by any external conditions, e.g. biological or chemical ones, which in turn might get caused because there is an obviously needed nerve cell not in place? Might these biological or chemical conditions get caused because neighbouring cells feel some stress and excrete some hormones?
Or might be the reason for new neurons to be created be caused by any neuro biological condition, though? Maybe because nerve cells divide when any of their offshoots -- axons, dendrites -- grew a "too large" tree//braid//knop? And, this rank growth divides itself from the remainder of the very nerve cell?
Or might it be that at some time there's no place left over on the main body of a neuron where any other neurons immediately can dock to, hence dendrites get started to grow? Or the docking nerve cells begin to grow axons, since these might fit between all the other dockers? Or is it that way, the nerve cell gets divided when there's no place left over on the core body of it?
PS.: I doubt there is any bird's view instance which decides whether or not to set up any new edge or cell (node). In other words, I doubt "the brain" decides that..anything at all what takes place within the brain itself//brain body, i.e. I doubt there is any other instance in brain but 'self' that makes any decisions regarding brain itself.
Updates: none so far
Sunday, June 24, 2007
Made the ancient postings accessibly by tags
Tagged the early postings of this blog to let it make provide more benefit, e.g. by enabling every reader to pick up topics by tags -- and then get all the posting on that very topic I posted here, so far.
Positive side-effect of that effort is that also for me, in future, it might become more easy for me to set up backward references to earlier posted articles and yet tackled subjects. Such as for thestill under-constructional posting on obstacles of traditional notion organizing systems MOM overcomes.
Updates: none so far
Positive side-effect of that effort is that also for me, in future, it might become more easy for me to set up backward references to earlier posted articles and yet tackled subjects. Such as for the
Updates: none so far
Weaknesses of Traditional Notion Organizing Systems
Weaknesses of traditional notion organizing systems:
In my opinion, they are wrong about when//with implying the item to the notion instead of just dealing with the idea of the item and vocabulary for the item: The idea, i.e. the immaterial variant, of a motorized vehicle of course is a part of, or at least is included to, the idea of a car.
Updates: none so far
- Traditional notion organizing systems aim at providing experts --
- people who already know the professional vocabulary and the items the vocabulary refers to
- people who are trained in using notion organizing systems
- The concept of notion traditional notion organizing systems make use of implies the real item too. Hence, in reality, notion organizing systems aren't organizing notions only, but also the items they refer to. -- That might be a reason for the omnipresent preference is a relationships over has a ones:
- Traditional notion organizing systems prefer is a relationships over has a ones: A car is a motorized vehicle, but a motorized vehicle is clearly not a part of a car. Therefore, a has a relationship to be established from car to motorized vehicle is wrong.
In my opinion, they are wrong about when//with implying the item to the notion instead of just dealing with the idea of the item and vocabulary for the item: The idea, i.e. the immaterial variant, of a motorized vehicle of course is a part of, or at least is included to, the idea of a car.
Updates: none so far
Friday, June 22, 2007
"big wet transistors" and "spaghetti wiring"
Doing the homework I caused myself, now have to do sent me back to the "10 Important Differences Between Brains and Computers" article of Chris Chatham I cross-read earlier today. His article reader Jonathan points out several weaknesses in Chris Chatham's argumentation. Although I consider him mostly right with his objections, I consider it nitpicking, mostly. In the end, I don't see the point he's about to make. Jonathan's arguing "[...] there must be some level of modularity occurring in the brain. My gut instinct is telling me here that a brain based completely on spaghetti wiring just wouldn't work very well..." obviously takes not into consideration that the single neurons themselves might be the entities of the brain that do the processing and that do constitute memory -- memory and processing in once. On this point, I am far from his arguments.
Another interesting point the reader Kurt van Etten puts into the round: "[...] (I do think a lot of writers equate neurons with big wet transistors)," -- Hm, I learned electrotechnics when in IT support assistant education, and every now and then I ponder about how to cast MOM nodes into hardware, but when doing so, I primarily think of the content storable by such a node. That I might make use of a transistor for that is a negligibility. -- I didn't think so far yet, but I don't presume to cast a MOM node into hardware to utilize transistors might be the only way. Anyway, interesting to learn like what the major part of people occupied by the topic might imagine a single neuron. ... Right now, I think that imagination might be a bit too simplified and doing so might lack this or that important property of a real neuron, hence anyone reducing their imagination of a single neuron to that simplicity might miss this or that important condition or might fail to get this or that insight, just because of a too restricted ("simplified") look at the matter.
... Well, I got up to comment number 18, but that one might need some deeper consideration. Hence, now I make a break and might continue with pondering that #18 comment later.
During reading the comments I opened some more links provided there, mostly by the commenters` names linking to these sites:
Updates: 20070623.12-42h CEST: added a headline to the posting
Another interesting point the reader Kurt van Etten puts into the round: "[...] (I do think a lot of writers equate neurons with big wet transistors)," -- Hm, I learned electrotechnics when in IT support assistant education, and every now and then I ponder about how to cast MOM nodes into hardware, but when doing so, I primarily think of the content storable by such a node. That I might make use of a transistor for that is a negligibility. -- I didn't think so far yet, but I don't presume to cast a MOM node into hardware to utilize transistors might be the only way. Anyway, interesting to learn like what the major part of people occupied by the topic might imagine a single neuron. ... Right now, I think that imagination might be a bit too simplified and doing so might lack this or that important property of a real neuron, hence anyone reducing their imagination of a single neuron to that simplicity might miss this or that important condition or might fail to get this or that insight, just because of a too restricted ("simplified") look at the matter.
... Well, I got up to comment number 18, but that one might need some deeper consideration. Hence, now I make a break and might continue with pondering that #18 comment later.
During reading the comments I opened some more links provided there, mostly by the commenters` names linking to these sites:
- Kurama's Secret Lab -- Blog destinado à discussão científica., looks Portuguese to me, which might cause me a hard time reading through it. However, there is a babelfish around, and also there might be this or that English posting amongst the others.
- Learning Computation -- A chronicle of one person's attempt to learn the theory of computation and related subjects.
- Greedy, Greedy Algorithms -- Talk of computation, mathematics, science, politics and all the associated philosophy from two guys with aspirations in the world of math. The current top postings of that blog don't look actually to be algorithm related at all, but interesting anyways. Information visualization, information freedom, and politics involved. However, probably not leading any further in the MOM issue.
Updates: 20070623.12-42h CEST: added a headline to the posting
Homework to do
My yesterday findings result in a lot of homework to do.
First of all, as a left-over of a former post I have to make clear what a MOM net is, what it stores and how it does so. The recent posting on the issue about finding a heater repair part by using a thesaurus is a first step there.
Second, Chris Chatham's posting on differences between brain and computers I reviewed. But I missed that there's a lot of reader comments I didn't read yet. A to do. Also the insight 'content representation' still seems to refer to marking up content by key words or thesaurus terms demands to make clear my point of view on that topic, and why I chose the term content representation rather than any other. And, in turn, what I refer to by "marking up content by key words" demands for a explanation. Things to do.
Third, what I already begun, is to tidy up the blog. I think posting might be much more useful if sudden readers can dive in at any point, without needing to know what I wrote about before. Therefore, from now on, key concepts shall be cleared tersely (hence the heater posting) by separate postings. And any new postings referring to these concepts shall do point there instead of going into detail every new posting -- which might disturb too much -- you as well as myself, when developing a thought.
Let`s see how it works out.
Updates: none so far
First of all, as a left-over of a former post I have to make clear what a MOM net is, what it stores and how it does so. The recent posting on the issue about finding a heater repair part by using a thesaurus is a first step there.
Second, Chris Chatham's posting on differences between brain and computers I reviewed. But I missed that there's a lot of reader comments I didn't read yet. A to do. Also the insight 'content representation' still seems to refer to marking up content by key words or thesaurus terms demands to make clear my point of view on that topic, and why I chose the term content representation rather than any other. And, in turn, what I refer to by "marking up content by key words" demands for a explanation. Things to do.
Third, what I already begun, is to tidy up the blog. I think posting might be much more useful if sudden readers can dive in at any point, without needing to know what I wrote about before. Therefore, from now on, key concepts shall be cleared tersely (hence the heater posting) by separate postings. And any new postings referring to these concepts shall do point there instead of going into detail every new posting -- which might disturb too much -- you as well as myself, when developing a thought.
Let`s see how it works out.
Updates: none so far
An Issue about Getting a Replacement for a Heater Part by Using a Thesaurus
One of the most ancient questions that led to MOM was the issue a former information science teacher of mine presented by one of the lectures he gave to us: He drafted the case someone had an issue with their heater and had to find the replacement part by using a thesaurus. Actually the task was the guy should look for the matching word in the thesaurus to order the part by mail.
Simply said, a thesaurus is a vocabulary with the aim to order that vocabulary. It helps experts to find the right words. Likely the 'thesaurus' embedded to any major text writing program does to lay people. The thesaurus relates the items it deals with to each other to bring them into a hierarchy: A mouse is a mammal, and a mammal is a vertebrate. The cat is a mammal too, so the cat node is placed next to the mouse one. I tiger is a cat too, as well as the lion and the panther, so they get placed as childs of the cat.
The core item the thesaurus deals with is a trinity of item, word for that item and thought item/imagination of the item. It's called a notion. The notion is an abstraction for an item, thus in every case is immaterial. Related to the state of matter the notion focuses on the imaginary part but never lets go the material part out of sight or the word. The thesaurus orders its words by looking at the words and the real items.
In a first step, words synonymous to each other get collected to a set. The most familiar one of those synonyms get picked and becomes declared to be the descriptor of of that set. If the descriptor is referred to, implicitly the whole set is meant -- or, thought-with.
In a second step, the thesaurus goes ahead to order the items, identified by their descriptors. Most widely used relationship between descriptors might be the is a relationship, just as demonstrated above: The cat is a mammal, the mammal is a vertebrate, and so on. Alternatively, another common relationship applied by thesauri is the has a relationship. It states that the vertebrate has a spine, and a human has a head, has a torso, has a pair of arms and also has a pair of legs and feet. However, the is a relationship is much more used but the has a one. Reason for that might be that the resulting network of relationships and descriptors would quickly become a rather dense graph, hardly to maintain.
Aside of these two kinds of relationship any creator of a thesaurus can set up any kind of relationship they might imagine. Such as associative relationships relating related notions to each other that cannot be brought together by using any other kind of relationships, such as cat and cat food. The available relationships may vary from thesaurus to thesaurus, as their developers might have chosen different kinds of relationships to use.
For the case of the heater, we assumed a thesaurus effectively consisting of is a relationships only, since that seems to be the most common set up of a thesaurus.
A thesaurus consisting of is a relationships only helps an expert to quickly find the words they already know. On the other hand, a lay person usually gets stuck in that professional slang rather quickly as they get unable to discern the one notion from the other. Thesauri traditionally don't aim at assisting lay people, so the definitions they provide for descriptors are barely more but a reminder -- as said, for something the thesaurus developers assume the user already knows. If the thesaurus provides that definition text at all. During my course of studies I learned, thesauri resemble deserts of words, providing definitions as rarely as deserts have oases.
So, the answer to the heater question is: Restricted to a thesaurus, the guy won't find the replacement part for his heater.
And the amazing part my information science teacher pointed to too, was that going to the next heating devices shop would solve the problem within a minute -- it would suffice if the guy would describe the missing part by its look.
That miracle stuck with me. I came to the point to wonder why not to set up kind of a thesaurus that would prefer has a over is a relationships and asked the teacher about that. I was pointed to issues of how to put that into practice? How to manage that heavily wired graph?
Well, that's a matter of coping with machines, so I went along, although I wasn't about to get any support from that teacher. On the other hand, I was familiar to programming since 1988 -- so what? ... And over time, MOM evolved.
Update: During tagging all the rather old postings which were already in this blog when blogger.com didn't offer post tagging yet, I noticed I presented another variant of the issue earlier, then related to a car replacement part.
Updates: 20070624: added reference to the car repair example
Simply said, a thesaurus is a vocabulary with the aim to order that vocabulary. It helps experts to find the right words. Likely the 'thesaurus' embedded to any major text writing program does to lay people. The thesaurus relates the items it deals with to each other to bring them into a hierarchy: A mouse is a mammal, and a mammal is a vertebrate. The cat is a mammal too, so the cat node is placed next to the mouse one. I tiger is a cat too, as well as the lion and the panther, so they get placed as childs of the cat.
The core item the thesaurus deals with is a trinity of item, word for that item and thought item/imagination of the item. It's called a notion. The notion is an abstraction for an item, thus in every case is immaterial. Related to the state of matter the notion focuses on the imaginary part but never lets go the material part out of sight or the word. The thesaurus orders its words by looking at the words and the real items.
In a first step, words synonymous to each other get collected to a set. The most familiar one of those synonyms get picked and becomes declared to be the descriptor of of that set. If the descriptor is referred to, implicitly the whole set is meant -- or, thought-with.
In a second step, the thesaurus goes ahead to order the items, identified by their descriptors. Most widely used relationship between descriptors might be the is a relationship, just as demonstrated above: The cat is a mammal, the mammal is a vertebrate, and so on. Alternatively, another common relationship applied by thesauri is the has a relationship. It states that the vertebrate has a spine, and a human has a head, has a torso, has a pair of arms and also has a pair of legs and feet. However, the is a relationship is much more used but the has a one. Reason for that might be that the resulting network of relationships and descriptors would quickly become a rather dense graph, hardly to maintain.
Aside of these two kinds of relationship any creator of a thesaurus can set up any kind of relationship they might imagine. Such as associative relationships relating related notions to each other that cannot be brought together by using any other kind of relationships, such as cat and cat food. The available relationships may vary from thesaurus to thesaurus, as their developers might have chosen different kinds of relationships to use.
For the case of the heater, we assumed a thesaurus effectively consisting of is a relationships only, since that seems to be the most common set up of a thesaurus.
A thesaurus consisting of is a relationships only helps an expert to quickly find the words they already know. On the other hand, a lay person usually gets stuck in that professional slang rather quickly as they get unable to discern the one notion from the other. Thesauri traditionally don't aim at assisting lay people, so the definitions they provide for descriptors are barely more but a reminder -- as said, for something the thesaurus developers assume the user already knows. If the thesaurus provides that definition text at all. During my course of studies I learned, thesauri resemble deserts of words, providing definitions as rarely as deserts have oases.
So, the answer to the heater question is: Restricted to a thesaurus, the guy won't find the replacement part for his heater.
And the amazing part my information science teacher pointed to too, was that going to the next heating devices shop would solve the problem within a minute -- it would suffice if the guy would describe the missing part by its look.
That miracle stuck with me. I came to the point to wonder why not to set up kind of a thesaurus that would prefer has a over is a relationships and asked the teacher about that. I was pointed to issues of how to put that into practice? How to manage that heavily wired graph?
Well, that's a matter of coping with machines, so I went along, although I wasn't about to get any support from that teacher. On the other hand, I was familiar to programming since 1988 -- so what? ... And over time, MOM evolved.
Update: During tagging all the rather old postings which were already in this blog when blogger.com didn't offer post tagging yet, I noticed I presented another variant of the issue earlier, then related to a car replacement part.
Updates: 20070624: added reference to the car repair example
Some articles on content representation
Since nearing the first mile stone of MOM SSC, I thought, it might make some sense to connect to others occupied with content representation. I technoratied for "content representation" (including the quotation marks) and found several postings aparently totally irrelated to content representation. Also, today "content representation" seems to primarily mean "mark up", e.g. by terms provided by a thesaurus or the like. However, I found one attracting me, pointing to another one which in turn pointed me to 10 Important Differences Between Brains and Computers by Chris Chatham, posted on March 27, 2007.
Number one of his list of differences is nothing new -- "Brains are analogue; computers are digital", therefore skipped.
Number two reveals a new buzz word for describing MOM, "content-addressable memory", and it describes it as follows: "the brain uses content-addressable memory, such that information can be accessed in memory through " [...] spreading activation" from closely related concepts. [...]" When I read it first, I thought, oh, there might be someone with a similar concept in mind like MOM. On the second look, I realized, that claim likely originates just from psychology. The review continues the above quote by "For example, thinking of the word 'fox' may automatically spread activation [...]" which points a bit into neurology. I wonder who that claim "thinking of a word" or "thinking of a fox" or even "thinking of the word 'fox'" can be proven to spin off activation. I mean, that would imply someone proved "the word 'fox'" and a neuron equal, since the neuron is that instance sending activation to other neurons. -- However, I share that opinion, the one a neuron represents an item, but I am just not aware of a proof for that. If you have such a one at hand, I'd be really glad if you could hint me to the source. (Just since it'd support my own claims.)
Aside, I don't share the idea thinking of a word might immediately stimulate "memories related to other clever animals" [as my source, the above linked article, continues] related content. I think, at least it needs to think of the fox itself instead of just the word "fox". And, to finish the quoted sentence, it ends in "fox-hunting horseback riders, or attractive members of the opposite sex."
Back to MOM, taking "content-addressable memory" as a label for it, actually is chosen accordingly: Chris Chatham continues his second difference with a "The end result is that your brain has a kind of 'built-in Google,' in which just a few cues (key words) are enough to cause a full memory to be retrieved." Well, that's exactly what MOM is after: To pick up matching "memories" by just a few cues. -- The way Chris Chatham is describing the issue is pretty close to the original issue that led me to figuring out MOM: A guy who got his heater damaged who must find the spare part by utilizing a thesaurus. The thesaurus mostly consists of abstraction relationships between item names listed there. And rather often, there is no definition for the items provided -- thesaurus makers seem to presume you're a specialist on that field of topic or you wouldn't make use of a thesaurus at all. However, restricted to that tool, if that tool is restricted to abstraction relationships mainly, you cannot find the part you need to repair the heater. But what if you'd remove all the is a (i.e. abstraction) relationships and set up a "kind of thesaurus" consisting of has a relationships only? -- That way, you'd find the spare part as quickly as your in-mind "Google" might do. At least if you've got another tool at hand that jumps you over all the crap of temporarily unnecessary details, like the knowledge that -- let's switch the example to a pet cat -- the four feet, torso, tail, neck and head that belong to the cat also belong to any quadruped animal. Such as a pet dog, or also a pet hamster.
With differences #3–#9 I were familiar with respectively became clear to me over the time I developed the Model of Meaning, e.g. the claim provided by "Difference # 6: No hardware/software distinction can be made with respect to the brain or mind". That's rather clear, but I am not going to explain it here, since this posting is just a note to me (and anyone who might be interested), that there is a posting around which by content is close to MOM.
Difference #10, on the first glance looked unfamiliar to me -- "Brains have bodies" --, but although I wasn't aware of those change blindness findings "that our visual memories are actually quite sparse" quickly brought me back to what I already know (well, strongly believe; I lack the laboratories to proove my theoretic findings by scissoring mice). It's rather clear that "the brain is 'offloading' its memory requirements to the environment in which it exists: why bother remembering the location of objects when a quick glance will suffice?"
Updates: none so far
Number one of his list of differences is nothing new -- "Brains are analogue; computers are digital", therefore skipped.
Number two reveals a new buzz word for describing MOM, "content-addressable memory", and it describes it as follows: "the brain uses content-addressable memory, such that information can be accessed in memory through " [...] spreading activation" from closely related concepts. [...]" When I read it first, I thought, oh, there might be someone with a similar concept in mind like MOM. On the second look, I realized, that claim likely originates just from psychology. The review continues the above quote by "For example, thinking of the word 'fox' may automatically spread activation [...]" which points a bit into neurology. I wonder who that claim "thinking of a word" or "thinking of a fox" or even "thinking of the word 'fox'" can be proven to spin off activation. I mean, that would imply someone proved "the word 'fox'" and a neuron equal, since the neuron is that instance sending activation to other neurons. -- However, I share that opinion, the one a neuron represents an item, but I am just not aware of a proof for that. If you have such a one at hand, I'd be really glad if you could hint me to the source. (Just since it'd support my own claims.)
Aside, I don't share the idea thinking of a word might immediately stimulate "memories related to other clever animals" [as my source, the above linked article, continues] related content. I think, at least it needs to think of the fox itself instead of just the word "fox". And, to finish the quoted sentence, it ends in "fox-hunting horseback riders, or attractive members of the opposite sex."
Back to MOM, taking "content-addressable memory" as a label for it, actually is chosen accordingly: Chris Chatham continues his second difference with a "The end result is that your brain has a kind of 'built-in Google,' in which just a few cues (key words) are enough to cause a full memory to be retrieved." Well, that's exactly what MOM is after: To pick up matching "memories" by just a few cues. -- The way Chris Chatham is describing the issue is pretty close to the original issue that led me to figuring out MOM: A guy who got his heater damaged who must find the spare part by utilizing a thesaurus. The thesaurus mostly consists of abstraction relationships between item names listed there. And rather often, there is no definition for the items provided -- thesaurus makers seem to presume you're a specialist on that field of topic or you wouldn't make use of a thesaurus at all. However, restricted to that tool, if that tool is restricted to abstraction relationships mainly, you cannot find the part you need to repair the heater. But what if you'd remove all the is a (i.e. abstraction) relationships and set up a "kind of thesaurus" consisting of has a relationships only? -- That way, you'd find the spare part as quickly as your in-mind "Google" might do. At least if you've got another tool at hand that jumps you over all the crap of temporarily unnecessary details, like the knowledge that -- let's switch the example to a pet cat -- the four feet, torso, tail, neck and head that belong to the cat also belong to any quadruped animal. Such as a pet dog, or also a pet hamster.
With differences #3–#9 I were familiar with respectively became clear to me over the time I developed the Model of Meaning, e.g. the claim provided by "Difference # 6: No hardware/software distinction can be made with respect to the brain or mind". That's rather clear, but I am not going to explain it here, since this posting is just a note to me (and anyone who might be interested), that there is a posting around which by content is close to MOM.
Difference #10, on the first glance looked unfamiliar to me -- "Brains have bodies" --, but although I wasn't aware of those change blindness findings "that our visual memories are actually quite sparse" quickly brought me back to what I already know (well, strongly believe; I lack the laboratories to proove my theoretic findings by scissoring mice). It's rather clear that "the brain is 'offloading' its memory requirements to the environment in which it exists: why bother remembering the location of objects when a quick glance will suffice?"
Updates: none so far
Thursday, June 21, 2007
Tagging The Links Might Reveal (The) Content Of Linked Web Pages
The 'link payload' -- tags assigned to a link, the users tag themselves with these tags when they click them, thus generating a cloud of tags of possible interests applying to the clicking individual user -- could be applied by a company that hosts its own site on its own server -- at least on a server it can set up the way they prefer.
In my previous example for such a link payload, I focused on a news company that provides a news overview consisting of nothing but the news articles headlines linking to the articles. That forces the users willing to read the article to click the link, thus tagging themselves by the tags assigned to that link. -- If the company provided the full text by RSS feed, they'd never learn the tag cloud the users would generate/reveal about themselves by clicking several such links.
Learning the interests of a current visitor in realtime might allow to pick more fitting ads to present.
Aside of that immediate advantage of tag based user tracking on a single site, what about the web? Aside of user tracking, a (tagged) headline link to a news article page reveals another particle of content, even without the chance to track the user at all: The link tags tag the linked page too. -- If there are multiple links pointing to the very page, a cloud of tags for that page cumulates. In other words, the page gets a content aside of the text written on that page: the tag cloud. Since that content is not present on that page, I'd call it content assigned to that page, not actually there. For short, maybe something like "content [assigned] to a page" instead of the familiar "content of/on a page".
One question is, whether content can be mined from the web immediately, without processing the text presented on the web. Mined in a way like determining tags for links, for users, for web pages.
One goal of processing the tag cloud assigned to a web page (or any other item, of course) might be to gather a MOM net, a condensed form of content. A multi-level directed graph storing distinct content by each of its nodes. I see, it might be helpful to go more into depth with this, explaining what a MOM net actually is and what it stores and how it does so.
I keep that in the back of my head for another post to come.
Updates: none so far
In my previous example for such a link payload, I focused on a news company that provides a news overview consisting of nothing but the news articles headlines linking to the articles. That forces the users willing to read the article to click the link, thus tagging themselves by the tags assigned to that link. -- If the company provided the full text by RSS feed, they'd never learn the tag cloud the users would generate/reveal about themselves by clicking several such links.
Learning the interests of a current visitor in realtime might allow to pick more fitting ads to present.
Aside of that immediate advantage of tag based user tracking on a single site, what about the web? Aside of user tracking, a (tagged) headline link to a news article page reveals another particle of content, even without the chance to track the user at all: The link tags tag the linked page too. -- If there are multiple links pointing to the very page, a cloud of tags for that page cumulates. In other words, the page gets a content aside of the text written on that page: the tag cloud. Since that content is not present on that page, I'd call it content assigned to that page, not actually there. For short, maybe something like "content [assigned] to a page" instead of the familiar "content of/on a page".
One question is, whether content can be mined from the web immediately, without processing the text presented on the web. Mined in a way like determining tags for links, for users, for web pages.
One goal of processing the tag cloud assigned to a web page (or any other item, of course) might be to gather a MOM net, a condensed form of content. A multi-level directed graph storing distinct content by each of its nodes. I see, it might be helpful to go more into depth with this, explaining what a MOM net actually is and what it stores and how it does so.
I keep that in the back of my head for another post to come.
Updates: none so far
Link payload to get an impression of user interests
Is it link payload? Or something like a content or a set of features the link clicking web users reveal about themselves?
Having a tool in reach that might mine immediately processable content from the web, the reorganizer module of MOM, I keep wondering how to actually mine the web.
Just the minute, I am skimming a news web site that, on its overview page, provides the headlines of the articles only. Not the least preview, not even a snipped of text, hinting on what the linked article mght deal with, and where it might dig into the depth. So, a human can say: If you click on that link, you might be interested in the topic spotlighted by the headline. Or, since I know the sometimes crudely set up headlines, there's a chance you clicked only to get an idea, what the heck the article might deal with. There's also the chance you'd click any link accidentally, but let's skip that possibility for now.
What I noticed the minute before, when I was skimming that headlines list was that converting the headline's words to nouns (e.g. by stemming) might suffice to tag the links. Given the case people would click only links they'd be interested in, in the mirror, any such link clicked reveals the topics the user is interested in -- the tags peel off the link and adhese to the person who clicked that link. In other words: By clicking the link, the users tag themselves. -- Track, what the user clicks over time, and you'd get not only a cloud of tags which you can link to a user, but by actually linking them to the user, applying reorganization, it's simple to learn the interests of a user. Add counting of the -- no, not of the links, as you might do for plain web site statistics, but instead -- add counting of the tags the users tag themselves with, and you might get a rather specific profile of the user. -- Cover a broad cloud of topics, thus a broad cloud of tags, and your users' profiles would become even sharper.
And, in the back of my head, there's still Google's advertising system. If each page, Google puts ads on, has to be 'enriched' by a handful of tags, visiting that page, the users tag themselves with those tags. If Google manages to assign that set of tags to individually you, Google might have quite a good impression of your interests.
Updates: none so far
Having a tool in reach that might mine immediately processable content from the web, the reorganizer module of MOM, I keep wondering how to actually mine the web.
Just the minute, I am skimming a news web site that, on its overview page, provides the headlines of the articles only. Not the least preview, not even a snipped of text, hinting on what the linked article mght deal with, and where it might dig into the depth. So, a human can say: If you click on that link, you might be interested in the topic spotlighted by the headline. Or, since I know the sometimes crudely set up headlines, there's a chance you clicked only to get an idea, what the heck the article might deal with. There's also the chance you'd click any link accidentally, but let's skip that possibility for now.
What I noticed the minute before, when I was skimming that headlines list was that converting the headline's words to nouns (e.g. by stemming) might suffice to tag the links. Given the case people would click only links they'd be interested in, in the mirror, any such link clicked reveals the topics the user is interested in -- the tags peel off the link and adhese to the person who clicked that link. In other words: By clicking the link, the users tag themselves. -- Track, what the user clicks over time, and you'd get not only a cloud of tags which you can link to a user, but by actually linking them to the user, applying reorganization, it's simple to learn the interests of a user. Add counting of the -- no, not of the links, as you might do for plain web site statistics, but instead -- add counting of the tags the users tag themselves with, and you might get a rather specific profile of the user. -- Cover a broad cloud of topics, thus a broad cloud of tags, and your users' profiles would become even sharper.
And, in the back of my head, there's still Google's advertising system. If each page, Google puts ads on, has to be 'enriched' by a handful of tags, visiting that page, the users tag themselves with those tags. If Google manages to assign that set of tags to individually you, Google might have quite a good impression of your interests.
Updates: none so far
Sunday, June 17, 2007
cluster your feed news: MOM reorganizer vs RSS feed news
The chance to reveal topics several different sources work with by applying a reorganizer also implies the chance to cluster RSS feeds by topic: Instead of approaching that issue by applying traditional information science procedures, alternatively the tags of the fetched articles could get looked up (retrieve the original article, pick its tags) and thrown through a reorganizer.
That might ease to skip feed news of usually valuable feeds on topics completely out of interest.
Updates: none so far
That might ease to skip feed news of usually valuable feeds on topics completely out of interest.
Updates: none so far
basic MOM peer scheme reworked
The recently recognized chance to build a MOM useful application without a need for a recognizer demands for a slight modification of the basic MOM peer scheme: 
Updates: none so far

Updates: none so far
MOM's reorganization could reveal topics/theme complexes
Currently, I am preparing to implement MOM's reorganization capabilities.
Today, some time amidst of it, I noticed that MOM could provide service already with only the reorganization functionality in place: Based on popular tagging, MOM [actually, its reorganizer] could reveal topics different sources (e.g. flickr photos or blog entries) deal with, unrecognizedly so far. -- The background:
Problem:
I've got lots of papers which are tagged. They deal with several different topics, on and off over time. There might be far later papers dealing with similar topics like any far earlier ones. -- Using the tags alone, doing that task intellectually, I might have a rather hard time: There are too many distinct tags to keep track of.
Approach for solution:
Reorganization could be applied: It might detect clouds of tags that belong together and 'mark' them by pooling them to separate new -- but yet unnamed -- 'tags' (= MOM nodes). That new tag, then, points to every paper the topic the tag represents deals with. -- That reduces the workload to be performed intellectually to find appropriate names for the newly created, first unnamed, tags. And, of course, to tag all those papers beforehand.
Benefit:
That does not only apply for my private issue of getting clear what topic I touched with MOM at what time, but also to any other unordered collection -- e.g. for papers collected in preparation of a diploma thesis..any scientific work, maybe even a law library..any library..all literature ever written.
Updates: none so far

Problem:
I've got lots of papers which are tagged. They deal with several different topics, on and off over time. There might be far later papers dealing with similar topics like any far earlier ones. -- Using the tags alone, doing that task intellectually, I might have a rather hard time: There are too many distinct tags to keep track of.
Approach for solution:
Reorganization could be applied: It might detect clouds of tags that belong together and 'mark' them by pooling them to separate new -- but yet unnamed -- 'tags' (= MOM nodes). That new tag, then, points to every paper the topic the tag represents deals with. -- That reduces the workload to be performed intellectually to find appropriate names for the newly created, first unnamed, tags. And, of course, to tag all those papers beforehand.
Benefit:
That does not only apply for my private issue of getting clear what topic I touched with MOM at what time, but also to any other unordered collection -- e.g. for papers collected in preparation of a diploma thesis..any scientific work, maybe even a law library..any library..all literature ever written.
Updates: none so far
Monday, June 11, 2007
Tag users
Google demands its adsense users to choose some trigger keywords/phrases. If they match a search of a Google user, the advertiser's ad might get displayed.
If the user clicks any such ad, they show interest in the topic of the ad, outlined by the trigger keywords. -- So, if one (or Google itself) were after to figure the interests of a user, Google could take these keywords and tag the user with them. -- The Google cookie one gets practically everywhere in the web, expiring in 2037, provides a unique handle for the user, for a long time. (And I really wouldn't wonder if they'd track 90% of all web users, individually.)
On the other hand, whenever a user enters a page featuring Google content, the user might become identified by their cookie. If Google is about to place ads on that site, by the cookie ID, Google immediately could figure the fields of interests of the visiting user. Also, immediately, it could update the user's record, since by visiting the page Google knew another bit about that user: He's likely interested in the topics dealt with on the page just entered. -- Which either the advertising real estate provider reveals by tagging its own page or which Google might do itself by traditional information science approaches.
Then, Google could select any ad that matches the user's profile and render it to the page currently being put out/the user is waiting for that it finishes rendering.
Is there a way to mimic that approach to gather tags of tags?
Updates: none so far
If the user clicks any such ad, they show interest in the topic of the ad, outlined by the trigger keywords. -- So, if one (or Google itself) were after to figure the interests of a user, Google could take these keywords and tag the user with them. -- The Google cookie one gets practically everywhere in the web, expiring in 2037, provides a unique handle for the user, for a long time. (And I really wouldn't wonder if they'd track 90% of all web users, individually.)
On the other hand, whenever a user enters a page featuring Google content, the user might become identified by their cookie. If Google is about to place ads on that site, by the cookie ID, Google immediately could figure the fields of interests of the visiting user. Also, immediately, it could update the user's record, since by visiting the page Google knew another bit about that user: He's likely interested in the topics dealt with on the page just entered. -- Which either the advertising real estate provider reveals by tagging its own page or which Google might do itself by traditional information science approaches.
Then, Google could select any ad that matches the user's profile and render it to the page currently being put out/the user is waiting for that it finishes rendering.
Is there a way to mimic that approach to gather tags of tags?
Updates: none so far
Tuesday, June 05, 2007
What is that, MOM SSC is after?
MOM SSC is after to get out a basic MOM peer, that can determine hidden content from a given MOM graph and recognize the presence of items by varying and not predictable patterns of features of these
items being given, e.g. signalled by sensors.
All of that on a strictly reviewable way, maybe even a laymen compatible one. It's avoiding every kind of black boxes such as self-organized neuronal networks especially when featuring loops.
Updates: 20070606.10-02h CEST: changed the last sentence to make more clear what I meant.

All of that on a strictly reviewable way, maybe even a laymen compatible one. It's avoiding every kind of black boxes such as self-organized neuronal networks especially when featuring loops.
Updates: 20070606.10-02h CEST: changed the last sentence to make more clear what I meant.
Labels:
FAVA,
goal of MOM SSC,
images,
MOM,
MOM peer,
peer to peer network,
Simple Set Core
docs are live!
The docs yesterday put into the MOM Simple Set Core framework, today go live. Here's a preview. Later, when gna.org's cron will have updated the MOM SSC website, the whole docs will get their own place. Up to then, I'd keep the preview link mentioned beforehand.
Matching the improved documentation, there's a new tag of the framework, now labeled v0.2.2.
Update: Okay, gna's cron made it to the MOM SSC site, thus, the docs are live and available by their official URL. Therefore removed the preview link above.
Updates: 20070605.14-46h CEST: mentioned the official site went live & removed the preview link.
Matching the improved documentation, there's a new tag of the framework, now labeled v0.2.2.
Update: Okay, gna's cron made it to the MOM SSC site, thus, the docs are live and available by their official URL. Therefore removed the preview link above.
Updates: 20070605.14-46h CEST: mentioned the official site went live & removed the preview link.
Sunday, June 03, 2007
new release: documentation improved, dotty output added
New version is out! It mainly features improved documentation of all of the files of the framework, but also introduced better MOM net output for MOMedgesSet and MOMnet class. For the latter even a simple dotty output method.
See here a sample MOM net created by MOMnet and rendered by dot:
Two layer MOM nets oftenly contain hidden, i.e. not explicit (i.e. implicit) content. To have a generator for them available constitutes the chance to develop a detector for such implicit content and to make it explicit. A mechanism that takes both of these steps is known as reorganizsation. -- Which might become implemented next.
Updates: none so far
See here a sample MOM net created by MOMnet and rendered by dot:

Updates: none so far
Friday, May 25, 2007
New version is out!
As before, the version is on gna.org, part of a subversion repository. Quick sum up of the changelog: What changed mostly is MOMnet.rb: Now it is validated and can generate MOM networks.
The option to get MOM networks to be generated relieves the developer to define -- and possibly debug -- MOM networks by his/her own. (I experienced, because of their dual nature -- edges meaning might be given/is given --, MOM networks are harder to debug but pure program code.) Having one -- better: more but one..multiple -- MOM network(s) at hand allows to reorganize the network to make implicit content explicit (marketing speak: "generate new content from yet given one/determine previously unknown content").
Other changes made possible to derive one HomogenousSetDerivatesInfrastructure class from another and inherit the class of the items to be dealed with too. Previously, that class was mentioned in a plain class variable of HomogenousSetDerivatesInfrastructure, which Ruby does not inherit normally. The recent change circumvents that Ruby default behaviour: MOMnet derives from MOMedgesSet, but only the latter becomes initialized to deal with MOMnetEdges only. MOMnet inherits that initialization from MOMnetEdge. -- Previously, there had been a need to tell every derived class explicitly which kind of items it should deal with although a developer would have expected the class to know that because of its anchestry/because its parent was for item class X, hence any child should be like that too. So, the change makes the framework meet that intuitive presumption: Now, children of HomogenousSetDerivatesInfrastructure know the class of the items to deal with.
A minor improvement was to add some output methods to the framework. Additionally, MOMedgesSet now can export to dotty, a graph visualization tool.
Last not least, I extracted changelogs from my local subversion repository for earlier check-ins to the public repository and added them to there too.
Updates: none so far
The option to get MOM networks to be generated relieves the developer to define -- and possibly debug -- MOM networks by his/her own. (I experienced, because of their dual nature -- edges meaning might be given/is given --, MOM networks are harder to debug but pure program code.) Having one -- better: more but one..multiple -- MOM network(s) at hand allows to reorganize the network to make implicit content explicit (marketing speak: "generate new content from yet given one/determine previously unknown content").
Other changes made possible to derive one HomogenousSetDerivatesInfrastructure class from another and inherit the class of the items to be dealed with too. Previously, that class was mentioned in a plain class variable of HomogenousSetDerivatesInfrastructure, which Ruby does not inherit normally. The recent change circumvents that Ruby default behaviour: MOMnet derives from MOMedgesSet, but only the latter becomes initialized to deal with MOMnetEdges only. MOMnet inherits that initialization from MOMnetEdge. -- Previously, there had been a need to tell every derived class explicitly which kind of items it should deal with although a developer would have expected the class to know that because of its anchestry/because its parent was for item class X, hence any child should be like that too. So, the change makes the framework meet that intuitive presumption: Now, children of HomogenousSetDerivatesInfrastructure know the class of the items to deal with.
A minor improvement was to add some output methods to the framework. Additionally, MOMedgesSet now can export to dotty, a graph visualization tool.
Last not least, I extracted changelogs from my local subversion repository for earlier check-ins to the public repository and added them to there too.
Updates: none so far
Thursday, May 24, 2007
new version in the queue
There's a new version of the MOM Ruby framework in the queue. :)
Updates: none so far
Updates: none so far
Sunday, April 08, 2007
upgrades on MOM SSC classes, introduction text
To several of the ruby MOM Simple Set Core classes, I added a demo program which aimed at showing the respect class works/aimed at crashing if there's a bug. Well, actually, the demo programs shall call each method with any possible value (including invalid ones). If the program runs trough, everything is okay; if it doesn't, that means homework, i.e. the class needs to be fixed. Yet a few days ago, I uploaded the upgraded classes to the MOM SSC repository (changelog).
I didn't change the version number, since the moment I want to do this, is when MOMnet.rb is completed also. -- That equals the moment, when all the classes using MOMnetNode and MOMnetConnection work.
Additionally, I took the time to improve (well, actually, replace) the description the goals of the MOM SSC project. I hope, now any passer-by can figure out the idea quite easy.
Updates: none so far
I didn't change the version number, since the moment I want to do this, is when MOMnet.rb is completed also. -- That equals the moment, when all the classes using MOMnetNode and MOMnetConnection work.
Additionally, I took the time to improve (well, actually, replace) the description the goals of the MOM SSC project. I hope, now any passer-by can figure out the idea quite easy.
Updates: none so far
Labels:
introduction,
MOM SSC framework,
Simple Set Core,
upgrades
Saturday, March 10, 2007
closely related work of MOM/FAVA
On an online search approach to get nailed down how terminologies, taxonomies, classification, thesauri (etc) related to each other, I noticed the work of the post-doctoral researcher Yannis Tzitzikas of the University of Namur (F.U.N.D.P.), Belgium. His research on faceted taxonomies, especially the application of them to peer to peer networks, looks very close to my MOM/FAVA research.
Updates: none so far
Updates: none so far
Tuesday, March 06, 2007
Released v0.1.0 of the drafts of the sources
Version 0.1.0 (initial_MOM_net_framework/) of the MOM net generator is there. As the previous ones, also this one is to be considered as drafts. Nothing functional yet.
Updates: none so far
Updates: none so far
Monday, March 05, 2007
Chances of the MOM project
Despite the MOM project does not strive for helping out neither the tagging approaches nor those of the Semantic Web movement, MOM probably can help both. And, other way round, both probably could provide some good to the MOM project.
Updates: none so far
Updates: none so far
MOM Simple Set Core project approved
The MOM Simple Set Core project at gna.org was approved on Wednesday, 28 Feb 2007.
On Sunday, 4 March 2007, the first bunch of files was uploaded.
Since a few minutes, it also features a serious project summary.
The project's website at gna.org is sported via the source code repository. A dummy project web page was checked in, recently, but it is not yet live. Possibly, because of the repository detour.
Updates: none so far
On Sunday, 4 March 2007, the first bunch of files was uploaded.
Since a few minutes, it also features a serious project summary.
The project's website at gna.org is sported via the source code repository. A dummy project web page was checked in, recently, but it is not yet live. Possibly, because of the repository detour.
Updates: none so far
Wednesday, February 21, 2007
About the Simple Set Core
The Simple Set Core project is about a set engine. Aim of this set engine is to recognize ("identify") items -- sets of features -- by only some of their features, to store these items and their features recursively as directed graphs, and to reorganize these graphs so that as well implicit items/features become visible as the graph as a whole becomes less dense, thus more easy to handle.
Updates: none so far
Background
The set engine is part of a larger project, the Model of Meaning. Its approach is that notions ("meanings") consist of smaller notions (or raw data like "light sensed"). Different but common approaches, the Model of Meaning drops the familiar "is a" relationship between things: The assumption of the model is that, despite a car is a kind of a vehicle, a vehicle is a part of the car. -- At first glance this is hard to comprehend, but isn't it so that you are just thinking of the car and the vehicle? Then, both are not physical, thus there is no physical problem of "cramming" a just imagined vehicle within a car. Which also is just imagination.Benefit For The Web
Having the Model of Meaning in mind, the set engine alone can do the web a big service: If tags would be related to other tags by "is part of" relationships, first, the tagging folks could quit to mention implications, second, the people searching for content could get the matching content even when looking for low level implications of the very content.Perspective
Also, if there is a way to recognize items by just parts of their features, tag graphs could be integrated with each other, automatically, simply because the items mentioned in the graphs could be recognized by their features also; and, dropping the tags, replacing them by notion identifiers ("IDs") where the tags become attached to, maybe that could overcome even the language barrier, once and for all.Updates: none so far
Now, MOM will be developed publicly
Just a few minutes ago, I queued the core set engine for approval as a GPL project at gna.org.
The long description of the SSC project, I'll file soon.
Updates: none so far
The long description of the SSC project, I'll file soon.
Updates: none so far
If word processors could know the words meanings...
If complex content get be built out of atomic pieces (of content?), how should the complex content become clear, ever?
I think about a word processor. The whole document becomes built out of words, and these out of letters. There is no machine understandable content attached to the words; the words remain just strings of letters. The machine has no idea about the meaning of these strings of letters nor of the sequences of words forming sentences and the whole document.
If at least the words had concepts attached, for a machine it might become much more simple to figure out the content of the whole document. Also since the words theirselves are somewhat related to each other by the underlaying grammar.
To get at least the content of the words accessible, an approach could be to integrate a request for explanation mechanism into the spell checker framework: If the content of the word typed-in is unknown, the user should explain it.
Updates: none so far
I think about a word processor. The whole document becomes built out of words, and these out of letters. There is no machine understandable content attached to the words; the words remain just strings of letters. The machine has no idea about the meaning of these strings of letters nor of the sequences of words forming sentences and the whole document.
If at least the words had concepts attached, for a machine it might become much more simple to figure out the content of the whole document. Also since the words theirselves are somewhat related to each other by the underlaying grammar.
To get at least the content of the words accessible, an approach could be to integrate a request for explanation mechanism into the spell checker framework: If the content of the word typed-in is unknown, the user should explain it.
Updates: none so far
Tuesday, February 20, 2007
What is MOM?
MOM is the acronym for "Model of Meaning". It is founded on one basic assumption, that a notion always consists out of simpler notions or, alternatively, out of raw data -- like "light sensored"
Like ontologies, the MOM building of notions is represented as a graph, a heavily wired network, where the notions make the nodes and the edges indicate which notion consists out of which other notions. Other but common ontologies, MOM avoids edges injecting knowledge which is unreachable to a system that gets the network as its knowledge base. For example, in thesauri there are edges like "this is an antonym to that" and "this a broader term to that". And most of the thesaurus edges (called "relationships") are abstracting. So, for example a dog node may be immediately attached to a animal node. But that implies: The system cannot sense why the dog may be an animal. It fully depends on the humans who foster the network. The same in the case of antonym or even more sophisticated relationships. -- As far as I know, most ontologies tend to make use of such knowledge injecting edges.
Related to this, there is one another big difference between MOM and common classifications/thesauri (also known as "controlled vocabularies"): MOM builds upon a slightly different definition of the term "notion".
Usually, notions are thought of as a triangle of term, item, and thought of the item. MOM does not focus on the item, nor does it have any interest on the terms. (Different cultures have different terms for the same items, hence why care?) Thus, the MOM nodes in fact don't represent notions but just thoughts of items. That makes a huge difference: Relying on the traditional definition of notions, a classification or a thesaurus can validly define a motor vehicle to consist of e.g. a set of wheels, engine, body (amongst others), but validly it can not define a van to consist of a motor vehicle and other parts, since a motor vehicle, simply, is not a part of a van. MOM, on the other hand, ignores the chance that a physical item might be attached to a notion. Hence, yes, part of a van may be a motor vehicle.
This insight allowed to drop the dominating kind of relationship of traditional controlled vocabularies, the abstraction relationship. Which MOM dropped in fact. Which led to a single remaining kind of edges: The partial one. Questions?
Updates: none so far
Like ontologies, the MOM building of notions is represented as a graph, a heavily wired network, where the notions make the nodes and the edges indicate which notion consists out of which other notions. Other but common ontologies, MOM avoids edges injecting knowledge which is unreachable to a system that gets the network as its knowledge base. For example, in thesauri there are edges like "this is an antonym to that" and "this a broader term to that". And most of the thesaurus edges (called "relationships") are abstracting. So, for example a dog node may be immediately attached to a animal node. But that implies: The system cannot sense why the dog may be an animal. It fully depends on the humans who foster the network. The same in the case of antonym or even more sophisticated relationships. -- As far as I know, most ontologies tend to make use of such knowledge injecting edges.
Related to this, there is one another big difference between MOM and common classifications/thesauri (also known as "controlled vocabularies"): MOM builds upon a slightly different definition of the term "notion".
Usually, notions are thought of as a triangle of term, item, and thought of the item. MOM does not focus on the item, nor does it have any interest on the terms. (Different cultures have different terms for the same items, hence why care?) Thus, the MOM nodes in fact don't represent notions but just thoughts of items. That makes a huge difference: Relying on the traditional definition of notions, a classification or a thesaurus can validly define a motor vehicle to consist of e.g. a set of wheels, engine, body (amongst others), but validly it can not define a van to consist of a motor vehicle and other parts, since a motor vehicle, simply, is not a part of a van. MOM, on the other hand, ignores the chance that a physical item might be attached to a notion. Hence, yes, part of a van may be a motor vehicle.
This insight allowed to drop the dominating kind of relationship of traditional controlled vocabularies, the abstraction relationship. Which MOM dropped in fact. Which led to a single remaining kind of edges: The partial one. Questions?
Updates: none so far
Sunday, February 18, 2007
To Keep One's Insights Secret is the Best Chance to Get Stuck.
I am working on a model to represent content in a manner free of words. There are two other main parts tightly related to it: To recognize content by a variable set of given features. And, to reorder the stored content so that implicitly represented content becomes explicit while the already explicit part becomes more straight at the same time.
There is one main thing I avoided during my approach: black boxes. Things that base on people's believes but on proven facts. Things that are overly complex, hence may be estimated only, but not proven.
I avoided two common approaches: utilizing artificial intelligence and linguistics.
Nevertheless, I continued the quest. There is software available, nowadays, hence why to keep the chance out of mind?
Over time, I came to the insight, that notions might be constituted by sets of features, mainly. There -- I had "words" represented. More precisely: the items which are treated as the "templates" for the notions. ... I begun to develop an algorithm to recognize items by their features, varying sets of features, effectively getting rid of the need for globally unique identifier numbers, "IDs" for short. I had a peer to peer network in mind, which automatically should exchange item data sets. That demanded for a way not to identify but at least to recognize items by their features.
Since items can be part of other items -- like a wheel can be part of a car, and a car a part of a car transporter --, I switched from sets to graphs. -- To make clear that the edges used by my model are not simple graph edges, but also to differentiate them from classification/thesauri relationships, I call them "connections".
Then I noticed similarities to neurological structures, mainly neurons, axons, dendrites. Also, I noticed, the yet developed tools could not represent a simple "not", e.g. "a not red car", I begun to experiment with meaning modifying edges. Keeping in mind, that there is probably no magical higher instance providing the neurons with knowledge -- as for example knowledge about that one item is the opposite of another --, I kept away from connections injecting new content/knowledge; in this case knowledge about how to interpret an antonym relationship. I strove for the smallest set of variations of connections.
However, even without content modifying connections, the tag phenomenon common to the web could take great benefit: The connections between the items make clear which item is implication of which other(s). Applied to tagging, users would not need to mention the implications anymore: The implications would be available by the underlaying ontology. (And the ontology could be enhanced by a central site, by individual users, or by a peer to peer network of users.)
But usually, every feature points to multiple items at once. Most probably, every item a feature is pointing to is chosen reasonably, i.e. the item the feature is pointing to is neither randomly chosen nor complete trash. Thus, a simple count might be enough: How many given features point to which items? How great is the quota of features pointing to a particular item, compared to the number of total incoming feature connections? -- The number of incoming feature connections, I call stimulations.
There's one condition applied to the recognition: If one node gets a particular number of stimulations, e.g. two stimulations, that very node will be considered to be "active" itself, hence stimulating its successor nodes as well. For a basic implementation of recognition, this approach is enough. A more sophisticated kind of recognition also considers nodes stimulated only, but not activated.
However, having recognition at hand -- even at this most basic level -- would finally support the above aproach of tagging to leave alone the implications: Given only a handful of features would be enough to determine the item meant. Also, applied to online search, the search engine could determine the x most probably meant items and include them into the search.
Despite these, I see one big chance: Currently, if a person gets a physical item the individual does not know and cannot identify, she or he needs to let it recognize by someone else. Usually your vendor. Where you have to move the part to. Some parts are heavy, others cannot be moved simply. And you are hindered using common tools to identify the object: Search engines don't operate on visual appearance, and information science tools like thesauri and classifications fail completely, simple because they prefer abstraction relationships over partial ones.
Using software able to recognize items by features would overcome such issues, completely, and independently of the kind of feature: It would be equal whether the feature would be a word, i.e. name, label, or color, shape, taste, smell or other. And, other but relational databases, there were no need for another table dimension for each feature -- just a unified method to attach new features to items.
Since the chains oftenly just can be cut down, I concentrated on the cases of unidentified implicit definitions. For simplicity, I imagined a set of features pointing to sets of items. Some of the features might point to the same items in common, e.g. the features <four legs>, <body>, <head>, and <tail> in common would point to the items <cat>, <dog>, and <elephant>. You might notice, that <cat>, <dog>, and <elephant>, all are animals, and also all these animals feature a body, four legs, head, and tail. Thus, <animal> is one implication of this set of features and items. The implication is not mentioned explicitely, but it's there.
Consequently, the whole partial network could be replaced by another one, mentioning the <animal> node as well: <four legs>, <body>, <head>, and <tail> would become features of the new <animal> node, and <animal> itself would become common feature of <cat>, <dog>, and <elephant>.
By that, the network would become more straight (since the number of connections needed would reduced from number of features * number of items to only number of features + number of items), hence also more lightweight. Also, items implied would become visible.
While this approach makes the implications visible, it opens two new doors: One, the identified implications cannot be named without the help of a human -- at least not easily. (Recognition could do a honour, but I skip that here.) The second issue is, that the newly introduced node ("<animal>") conflicts with recognition: For example: If there was a another node, e.g. <meows>, directly pointing to the <cat> node, after the reorganization, a given set of <meows> and <head>, only, would not result in <cat> anymore since each of the given features would, yes, stimulate their successor nodes, but not activate. -- To actively receive stimulations from predecessor nodes could be a solution, but I am not yet quite sure. As mentioned initially, this is a work in progress.
However, reorganization would automate identification of implications. People could provide labels for the added implication nodes. -- Which induces another effect of the model.
Instead, all the items and the features (whereby "features" is just another name for items located a level below the level of items initially considered) get identified by [locally only] unique IDs. The names of the items I'd store somewhere else, so that item names in multiple languages could point to the very item. That helps in localization of the system, but also, it opens the chance to overcome an issue dictionaries cannot manage: There are languages that do not provide an immediate translation for a given word -- because the underlaying concept is different. The English "to round out" term and the German "abrunden" is such an example: In fact, the German variant considers an external point of view ("to round by taking something away"), while the English obviously takes an internal one.
Not sticking on labels but on items/notions, the model features a chance to label the appropriate, maybe even slightly dismatching, nodes: The need to label exactly the same -- but probably not exactly matching -- node is gone. -- In a word: I think, in different cultures many notions differ slightly from similar ones of other cultures, but each culture labels only their own notions, ignoring slightly different points of view of other cultures. -- This notions/labeling issue, I imagine as puddles: Each culture has its own puddles for each of its notions. From the point of view of different languages, some puddles match greatly, maybe even totally, but some feature no intersection at all. Those are the terms having no counterpart in the other language.
In the long term, I consider the approach to build upon notions/items -- but on words -- as a real chance to overcome the language barrier.
Updates: none so far
There is one main thing I avoided during my approach: black boxes. Things that base on people's believes but on proven facts. Things that are overly complex, hence may be estimated only, but not proven.
I avoided two common approaches: utilizing artificial intelligence and linguistics.
Representing The Content
On dealing with thesauri and classifications, I noticed the fact that those ontologies force abstraction relationships between mentioned notions. Therefore, I thought about the alternative, to force the partial relationship. What would result, if all the notions had to be represented as partial relationships? -- A heavily wired graph. -- Originally, thesauri and classifications were fostered manually. Therefore, it looked clear to me, noone would like to foster a densely wired graph to keep track of notions.Nevertheless, I continued the quest. There is software available, nowadays, hence why to keep the chance out of mind?
Over time, I came to the insight, that notions might be constituted by sets of features, mainly. There -- I had "words" represented. More precisely: the items which are treated as the "templates" for the notions. ... I begun to develop an algorithm to recognize items by their features, varying sets of features, effectively getting rid of the need for globally unique identifier numbers, "IDs" for short. I had a peer to peer network in mind, which automatically should exchange item data sets. That demanded for a way not to identify but at least to recognize items by their features.
Since items can be part of other items -- like a wheel can be part of a car, and a car a part of a car transporter --, I switched from sets to graphs. -- To make clear that the edges used by my model are not simple graph edges, but also to differentiate them from classification/thesauri relationships, I call them "connections".
Then I noticed similarities to neurological structures, mainly neurons, axons, dendrites. Also, I noticed, the yet developed tools could not represent a simple "not", e.g. "a not red car", I begun to experiment with meaning modifying edges. Keeping in mind, that there is probably no magical higher instance providing the neurons with knowledge -- as for example knowledge about that one item is the opposite of another --, I kept away from connections injecting new content/knowledge; in this case knowledge about how to interpret an antonym relationship. I strove for the smallest set of variations of connections.
However, even without content modifying connections, the tag phenomenon common to the web could take great benefit: The connections between the items make clear which item is implication of which other(s). Applied to tagging, users would not need to mention the implications anymore: The implications would be available by the underlaying ontology. (And the ontology could be enhanced by a central site, by individual users, or by a peer to peer network of users.)
Recognizing The Content
Having that peer to peer network in mind, I needed a way to identify items stored at untrusted remote hosts. I noticed, that collecting sets of features together which theirselves would be considered to be items, meant nothing but a definition of the items by their features. Different peers -- precisely: users of the peer software -- might define the same items by different features. Which might leave only some of the features locally known/set to match those remotely set. -- However, most time that's enough to recognize an item by its features: Some features point to a single item only. These features are valuable: They are specific for that very item. If one of these specific features is given, most probably the item, the feature is pointing to, is meant.But usually, every feature points to multiple items at once. Most probably, every item a feature is pointing to is chosen reasonably, i.e. the item the feature is pointing to is neither randomly chosen nor complete trash. Thus, a simple count might be enough: How many given features point to which items? How great is the quota of features pointing to a particular item, compared to the number of total incoming feature connections? -- The number of incoming feature connections, I call stimulations.
There's one condition applied to the recognition: If one node gets a particular number of stimulations, e.g. two stimulations, that very node will be considered to be "active" itself, hence stimulating its successor nodes as well. For a basic implementation of recognition, this approach is enough. A more sophisticated kind of recognition also considers nodes stimulated only, but not activated.
However, having recognition at hand -- even at this most basic level -- would finally support the above aproach of tagging to leave alone the implications: Given only a handful of features would be enough to determine the item meant. Also, applied to online search, the search engine could determine the x most probably meant items and include them into the search.
Despite these, I see one big chance: Currently, if a person gets a physical item the individual does not know and cannot identify, she or he needs to let it recognize by someone else. Usually your vendor. Where you have to move the part to. Some parts are heavy, others cannot be moved simply. And you are hindered using common tools to identify the object: Search engines don't operate on visual appearance, and information science tools like thesauri and classifications fail completely, simple because they prefer abstraction relationships over partial ones.
Using software able to recognize items by features would overcome such issues, completely, and independently of the kind of feature: It would be equal whether the feature would be a word, i.e. name, label, or color, shape, taste, smell or other. And, other but relational databases, there were no need for another table dimension for each feature -- just a unified method to attach new features to items.
Reorganizing The Content
Also directly related to that peer to peer network in mind, peers exchanging item data sets -- e.g. nodes plus context (connections and attached neighbor nodes) -- could result in heavy wiring, superfluous chains of single-feature-single-item definitions, and lots of unidentified implicit item definitions. That needs to be avoided.Since the chains oftenly just can be cut down, I concentrated on the cases of unidentified implicit definitions. For simplicity, I imagined a set of features pointing to sets of items. Some of the features might point to the same items in common, e.g. the features <four legs>, <body>, <head>, and <tail> in common would point to the items <cat>, <dog>, and <elephant>. You might notice, that <cat>, <dog>, and <elephant>, all are animals, and also all these animals feature a body, four legs, head, and tail. Thus, <animal> is one implication of this set of features and items. The implication is not mentioned explicitely, but it's there.
Consequently, the whole partial network could be replaced by another one, mentioning the <animal> node as well: <four legs>, <body>, <head>, and <tail> would become features of the new <animal> node, and <animal> itself would become common feature of <cat>, <dog>, and <elephant>.
By that, the network would become more straight (since the number of connections needed would reduced from number of features * number of items to only number of features + number of items), hence also more lightweight. Also, items implied would become visible.
While this approach makes the implications visible, it opens two new doors: One, the identified implications cannot be named without the help of a human -- at least not easily. (Recognition could do a honour, but I skip that here.) The second issue is, that the newly introduced node ("<animal>") conflicts with recognition: For example: If there was a another node, e.g. <meows>, directly pointing to the <cat> node, after the reorganization, a given set of <meows> and <head>, only, would not result in <cat> anymore since each of the given features would, yes, stimulate their successor nodes, but not activate. -- To actively receive stimulations from predecessor nodes could be a solution, but I am not yet quite sure. As mentioned initially, this is a work in progress.
However, reorganization would automate identification of implications. People could provide labels for the added implication nodes. -- Which induces another effect of the model.
Overcome the Language Barrier
I mentioned that I kept away from connections injecting knowledge unreachable to the software. That's not all. I strive to completely avoid any kind of content unreachable, needing any external mind/knowledge storage which would provide interpretation of such injected content/knowledge. Hence, I also avoided to operate on a basis of labels for the items.Instead, all the items and the features (whereby "features" is just another name for items located a level below the level of items initially considered) get identified by [locally only] unique IDs. The names of the items I'd store somewhere else, so that item names in multiple languages could point to the very item. That helps in localization of the system, but also, it opens the chance to overcome an issue dictionaries cannot manage: There are languages that do not provide an immediate translation for a given word -- because the underlaying concept is different. The English "to round out" term and the German "abrunden" is such an example: In fact, the German variant considers an external point of view ("to round by taking something away"), while the English obviously takes an internal one.
Not sticking on labels but on items/notions, the model features a chance to label the appropriate, maybe even slightly dismatching, nodes: The need to label exactly the same -- but probably not exactly matching -- node is gone. -- In a word: I think, in different cultures many notions differ slightly from similar ones of other cultures, but each culture labels only their own notions, ignoring slightly different points of view of other cultures. -- This notions/labeling issue, I imagine as puddles: Each culture has its own puddles for each of its notions. From the point of view of different languages, some puddles match greatly, maybe even totally, but some feature no intersection at all. Those are the terms having no counterpart in the other language.
In the long term, I consider the approach to build upon notions/items -- but on words -- as a real chance to overcome the language barrier.
Conclusion
Despite the option of dropping the custom to label different items by the same name (as thesauri tend to do to reduce foster effort) and the possible long-term chance of overcoming the language barrier, I see three main benefits for the web, mainly for tagging and online search:- Tagging could be reduced to the core tags; all implications could be left to the underlaying ontology.
- Based on the same ontology, during search, sets of keywords could be examined to "identify" the items most probably meant. The same approach could be applied by the peer to peer network to exchange item data sets.
- Finally, the reorganization would keep the ontology graph lightweight, therefore ease the fostering. Also, the auto-detection of implications would support users in keeping their tags clear and definite. That could reduce blur in tag choose, thus increase precision in search/search results.
Updates: none so far
Subscribe to:
Posts (Atom)